Core Project 4 aims at converting the collected data into robotic actions in the fields, exploiting digital avatars. Precise robotic weeding, for example, seeks to intervene in a minimally invasive way, reducing the amount of inputs such as herbicides. This project develops autonomous field aerial and ground robots that detect and identify individual plants, weed mapping the field to treat individual plants with the most appropriate intervention. The robots precisely apply nitrogen fertilizer enabled by digital avatars that predict the plant nutrient demand and probable losses in the field.
Research Videos

Developing New Algorithms for Autonomous Decision Making to Optimize Robotic Farming
PhenoRob Junior Research Group Leader Marija Popovic talks about her research within Core Project 4: Autonomous In-Field Intervention.

Virtual Temporal Samples for RNNs: applied to semantic segmentation in agriculture
Normally, to train a recurrent neural network (RNN), labeled samples from a video (temporal) sequence are required which is laborious and has stymied work in this direction. By generating virtual temporal samples, we demonstrate that it is possible to train a lightweight RNN to perform semantic segmentation on two challenging agricultural datasets. full text in arxiv: https://arxiv.org/abs/2106.10118 check My GitHub for interesting ROS-based projects: https://github.com/alirezaahmadi
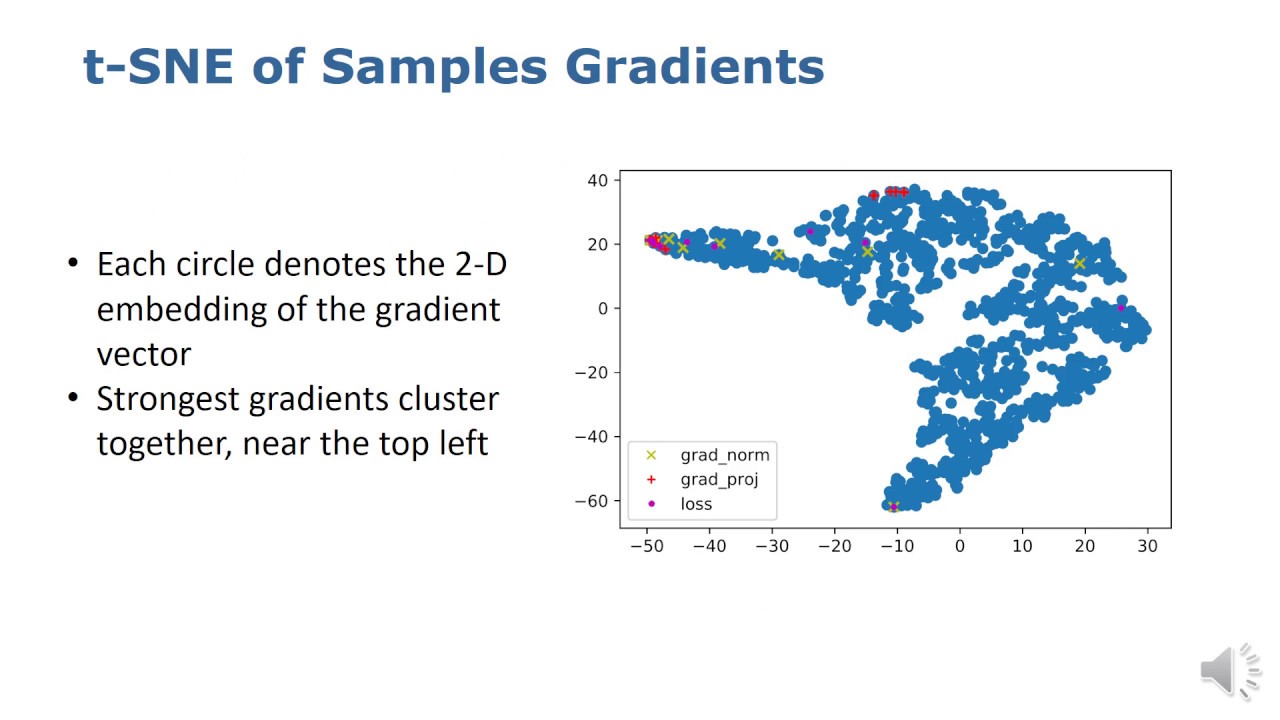
Talk by R. Sheikh on Gradient and Log-based Active Learning for Semantic Segmentation… (ICRA’20)
ICRA 2020 talk about the paper: R. Sheikh, A. Milioto, P. Lottes, C. Stachniss, M. Bennewitz, and T. Schultz, “Gradient and Log-based Active Learning for Semantic Segmentation of Crop and Weed for Agricultural Robots,” in Proceedings of the IEEE Int. Conf. on Robotics & Automation (ICRA), 2020. PDF: https://www.ipb.uni-bonn.de/wp-content/papercite-data/pdf/sheikh2020icra.pdf
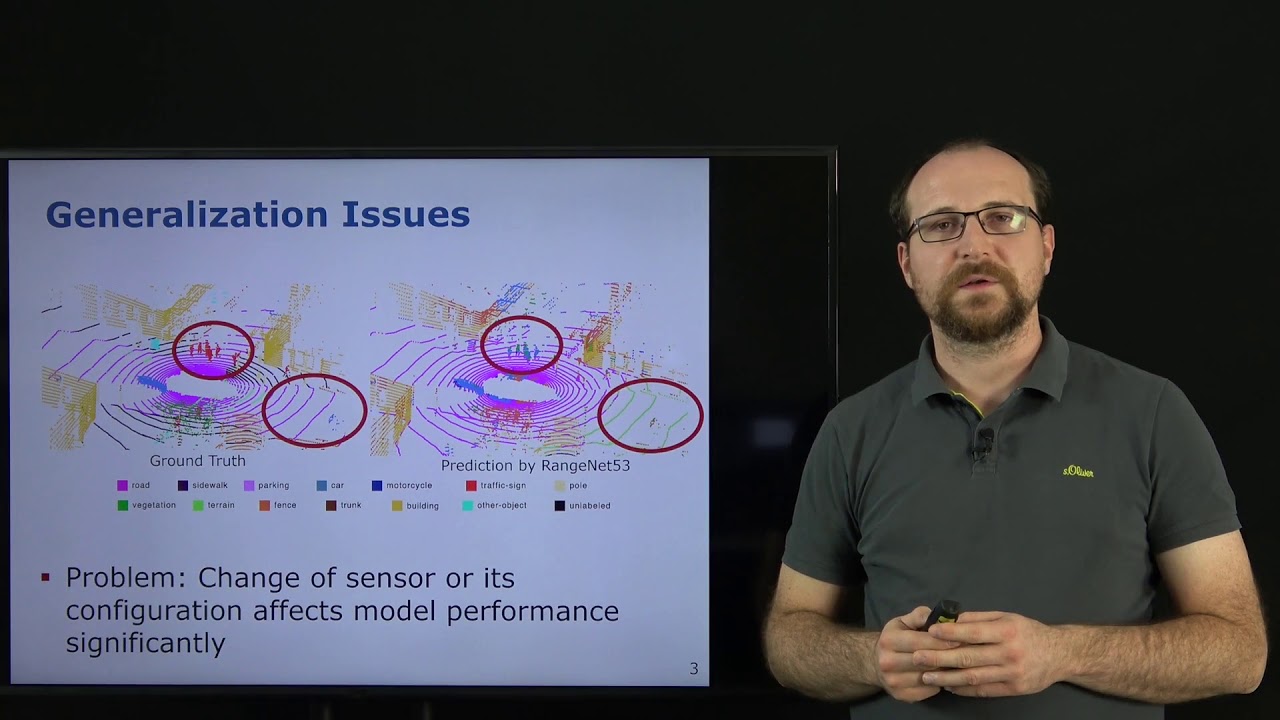
IROS’20: Domain Transfer for Semantic Segmentation of LiDAR Data using DNNs presented by J. Behley
F. Langer, A. Milioto, A. Haag, J. Behley, and C. Stachniss, “Domain Transfer for Semantic Segmentation of LiDAR Data using Deep Neural Networks,” in Proceedings of the IEEE/RSJ Int. Conf. on Intelligent Robots and Systems (IROS), 2020. Paper: https://www.ipb.uni-bonn.de/wp-content/papercite-data/pdf/langer2020iros.pdf

ICRA’2020: Visual Servoing-based Navigation for Monitoring Row-Crop Fields
Visual Servoing-based Navigation for Monitoring Row-Crop Fields by A. Ahmadi et al. In Proc. of the IEEE Intl. Conf. on Robotics & Automation (ICRA) , 2020.
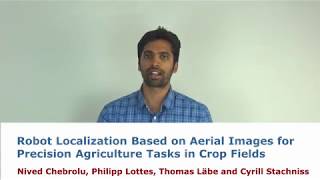
ICRA’19: Robot Localization Based on Aerial Images for Precision Agriculture by Chebrolu et al.
Robot Localization Based on Aerial Images for Precision Agriculture Tasks in Crop Fields by N. Chebrolu, P. Lottes, T. Laebe, and C. Stachniss In Proc. of the IEEE Intl. Conf. on Robotics & Automation (ICRA) , 2019. Paper: http://www.ipb.uni-bonn.de/wp-content/papercite-data/pdf/chebrolu2019icra.pdf
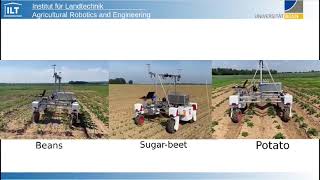
Towards Autonomous Visual Navigation in Arable Fields
Rou pare can be found in Arxiv at: [Towards Autonomous Crop-Agnostic Visual Navigation in Arable Fields](https://arxiv.org/abs/2109.11936) You can find the implementation in : [visual-multi-crop-row-navigation](https://github.com/Agricultural-Robotics-Bonn/visual-multi-crop-row-navigation) more detail about our project BonnBot-I and Phenorob at: https://www.phenorob.de/ http://agrobotics.uni-bonn.de/
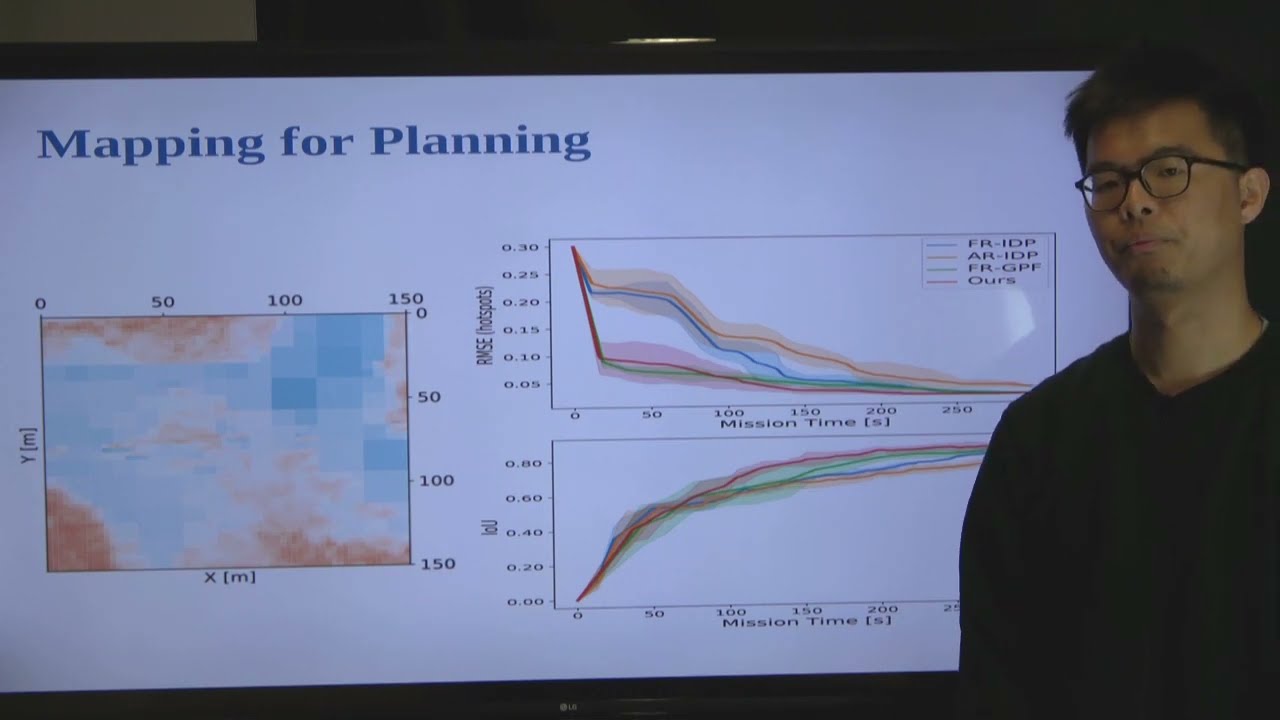
Adaptive-Resolution Field Mapping Using Gaussian Process Fusion With Integral Kernels by L.Jin et al
This short paper trailer is based on the following publication: L. Jin, J. Rückin, S. H. Kiss, T. Vidal-Calleja, and M. Popović, “Adaptive-Resolution Field Mapping Using Gaussian Process Fusion With Integral Kernels,” IEEE Robotics and Automation Letters, vol. 7, pp. 7471-7478, 2022. doi:10.1109/LRA.2022.3183797
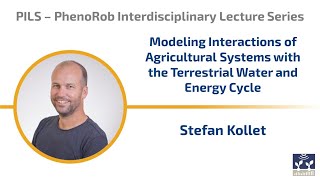
Modeling Interactions of Agricultural Systems with the Terrestrial Water and Energy Cycle
Stefan Kollet, Professor of Integrated Modeling of Terrestrial Systems at the Institute of Bio- and Geosciences (IBG-3), Forschungszentrum Jülich and at the Institute of Geosciences, University of Bonn gives a PhenoRob Interdisciplinary Lecture [PILS] on modeling interactions of agricultural systems with the terrestrial water and energy cycle.
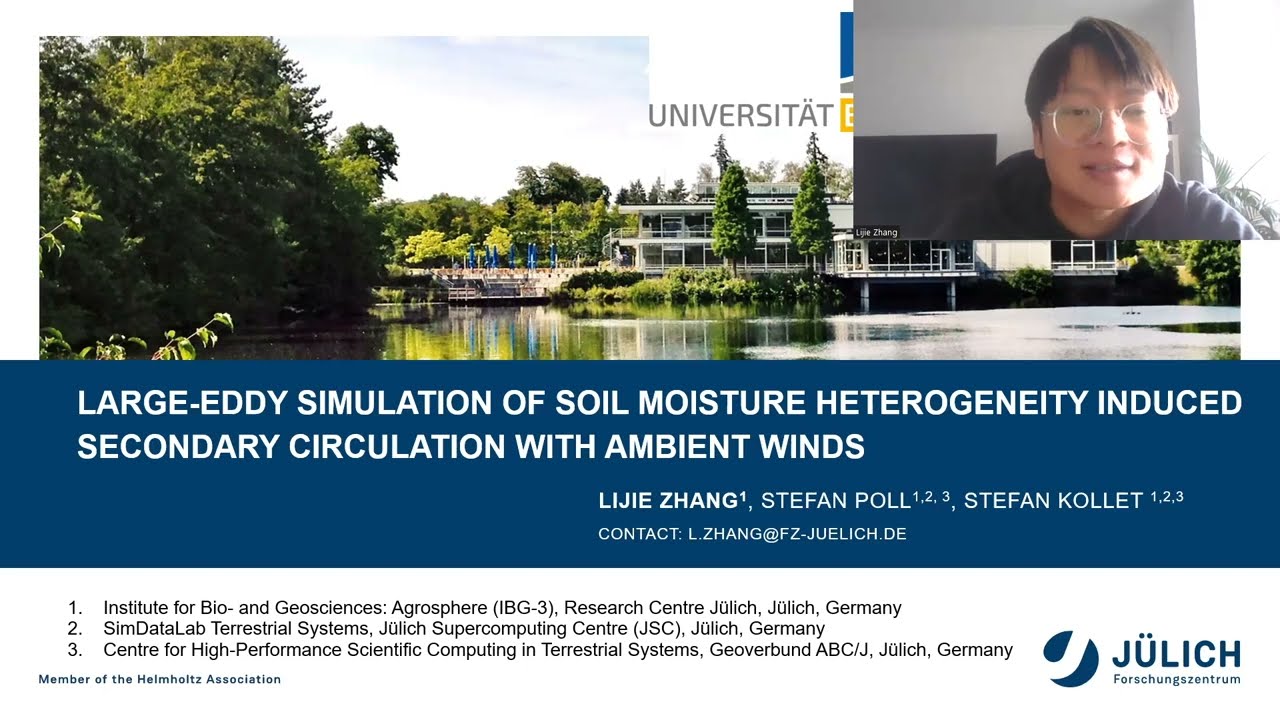
Large-eddy Simulation of Soil Moisture Heterogeneity Induced Secondary Circulation with Ambient Wind
This video is based on the following publication: L. Zhang, S. Poll, and S. Kollet, “Large-eddy Simulation of Soil Moisture Heterogeneity Induced Secondary Circulation with Ambient Winds,” Quarterly Journal of the Royal Meteorological Society, 2022. doi:10.5194/egusphere-egu22-5533
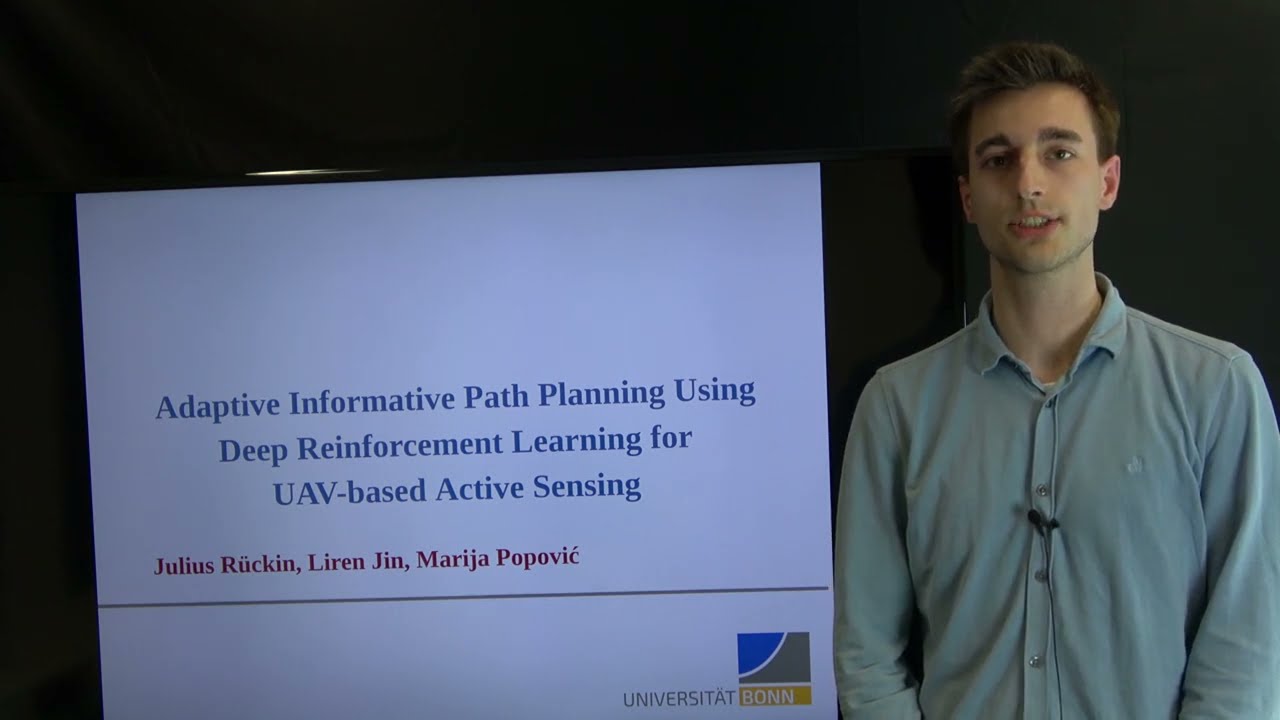
ICRA22: Adaptive Informative Path Planning Using Deep RL for UAV-based Active Sensing, Rückin et al.
Rückin J., Jin, L., and Popović, M., “Adaptive Informative Path Planning Using Deep RL for UAV-based Active Sensing,” in Proc. of the IEEE Intl. Conf. on Robotics & Automation (ICRA), 2022. doi: ICRA46639.2022.9812025 PDF: https://arxiv.org/abs/2109.13570 Code: https://github.com/dmar-bonn/ipp-rl
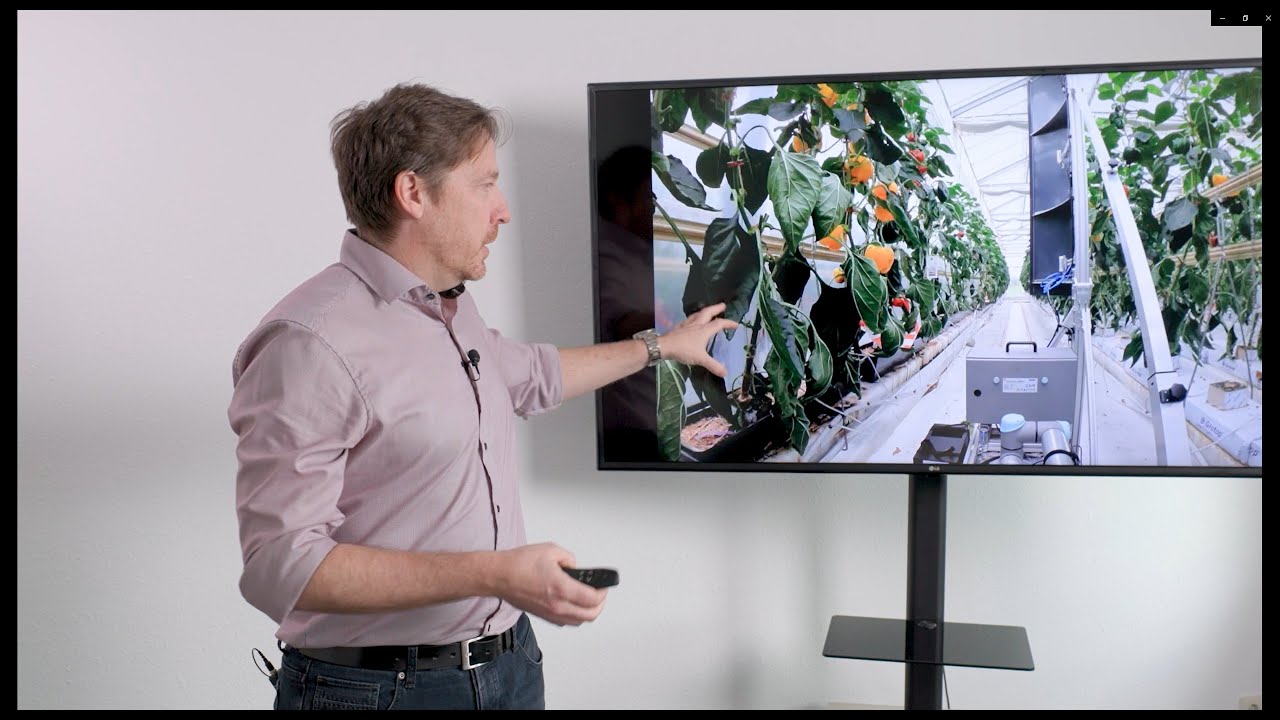
Crop Agnostic Monitoring Using Deep Learning
Prof. Dr. Chris McCool is Professor of Applied Computer Vision and Robotic Vision, head of the Agricultural Robotics and Engineering department at the University of Bonn M. Halstead, A. Ahmadi, C. Smitt, O. Schmittmann, C. McCool, “Crop Agnostic Monitoring Driven by Deep Learning”, in Front. Plant Sci. 12:786702. doi: 10.3389/fpls.2021.786702
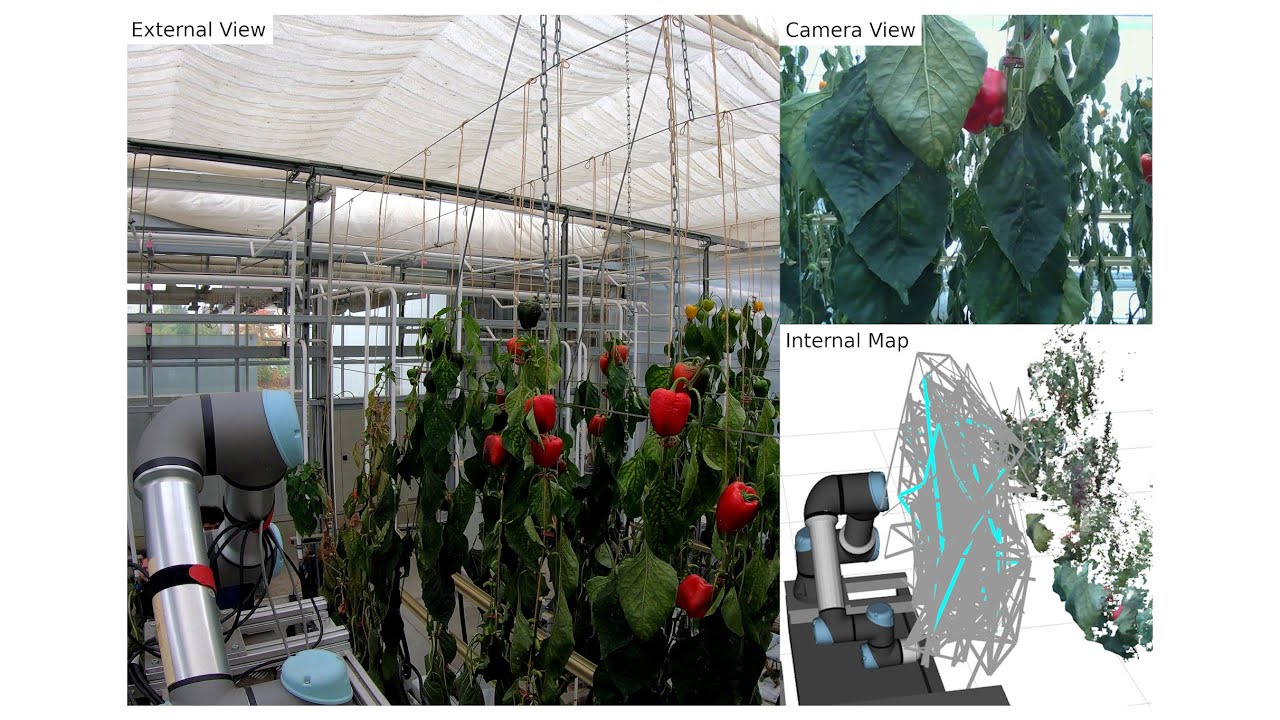
Graph-based View Motion Planning for Fruit Detection
This video demonstrates the work presented in our paper “Graph-based View Motion Planning for Fruit Detection” by T. Zaenker, J. Rückin, R. Menon, M. Popović, and M. Bennewitz, submitted to the International Conference on Intelligent Robots and Systems (IROS), 2023. Paper link: https://arxiv.org/abs/2303.03048 The view motion planner generates view pose candidates from targets to find new and cover partially detected fruits and connects them to create a graph of efficiently reachable and information-rich poses. That graph is searched to obtain the path with the highest estimated information gain and updated with the collected observations to adaptively target new fruit clusters. Therefore, it can explore segments in a structured way to optimize fruit coverage with a limited time budget. The video shows the planner applied in a commercial glasshouse environment and in a simulation designed to mimic our real-world setup, which we used to evaluate the performance. Code: https://github.com/Eruvae/view_motion_planner
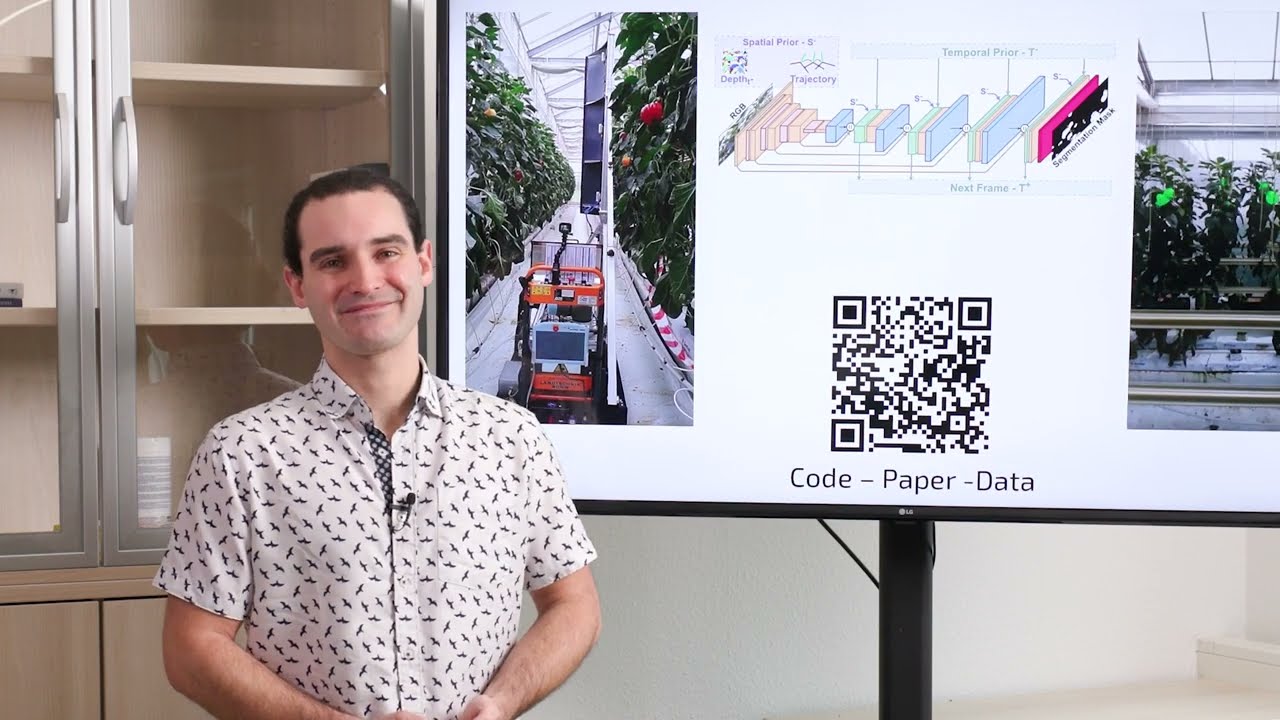
Explicitly Incorporating Spatial Information to Recurrent Networks for Agriculture
Claus Smitt is PhD Student at the Agricultural Robotics & Engineering department of the University of Bonn. Smitt, C., Halstead, M., Ahmadi, A., and McCool, C. , “Explicitly incorporating spatial information to recurrent networks for agriculture”. IEEE Robotics and Automation Letters, 7(4), 10017-10024. doi: 10.48550/arXiv.2206.13406
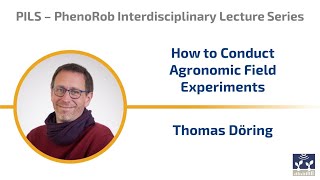
How to Conduct Agronomic Field Experiments
Thomas Döring, Professor of Agroecology and Organic Farming, Institute of Crop Science and Resource Conservation (INRES) at the University of Bonn gives a PhenoRob Interdisciplinary Lecture [PILS] on the topic of how to conduct agronomic field experiments.
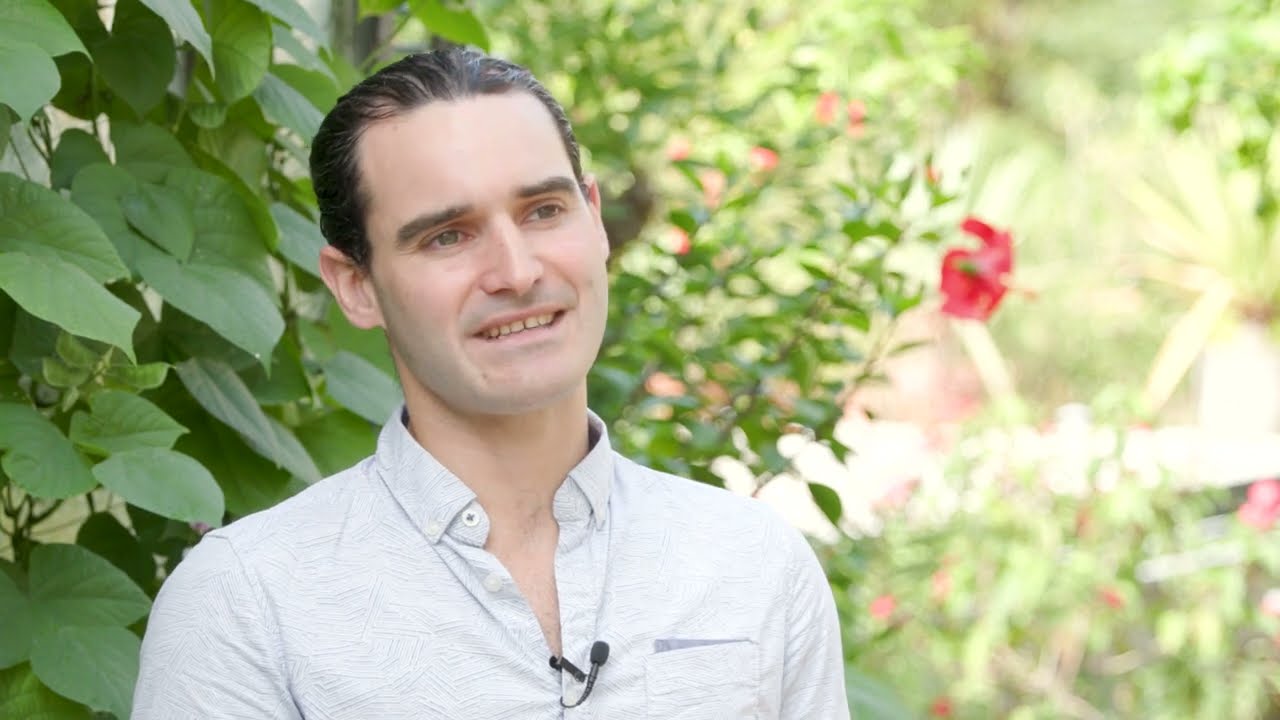
Faces of PhenoRob: Claus Smitt
In Faces of PhenoRob, we introduce you to some of PhenoRob’s many members: from senior faculty to PhDs, this is your chance to meet them all and learn more about the work they do. In this video you’ll meet Claus Smitt, a PhD student in the Agricultural Robotics and Engineering Group at the University of Bonn.
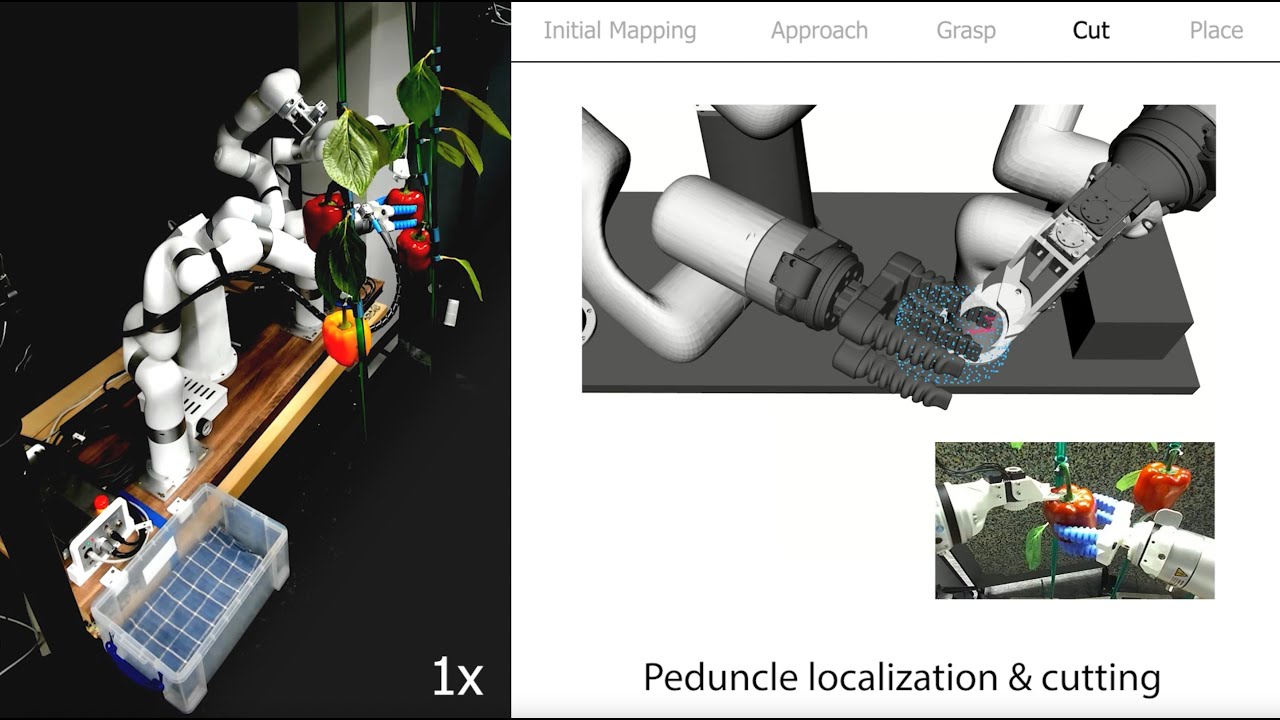
HortiBot: An Adaptive Multi-Arm System for Robotic Horticulture of Sweet Peppers, IROS’24 Submission
Paper Trailer for “HortiBot: An Adaptive Multi-Arm System for Robotic Horticulture of Sweet Peppers” by Christian Lenz, Rohit Menon, Michael Schreiber, Melvin Paul Jacob, Sven Behnke, and Maren Bennewitz submitted to IROS 2024
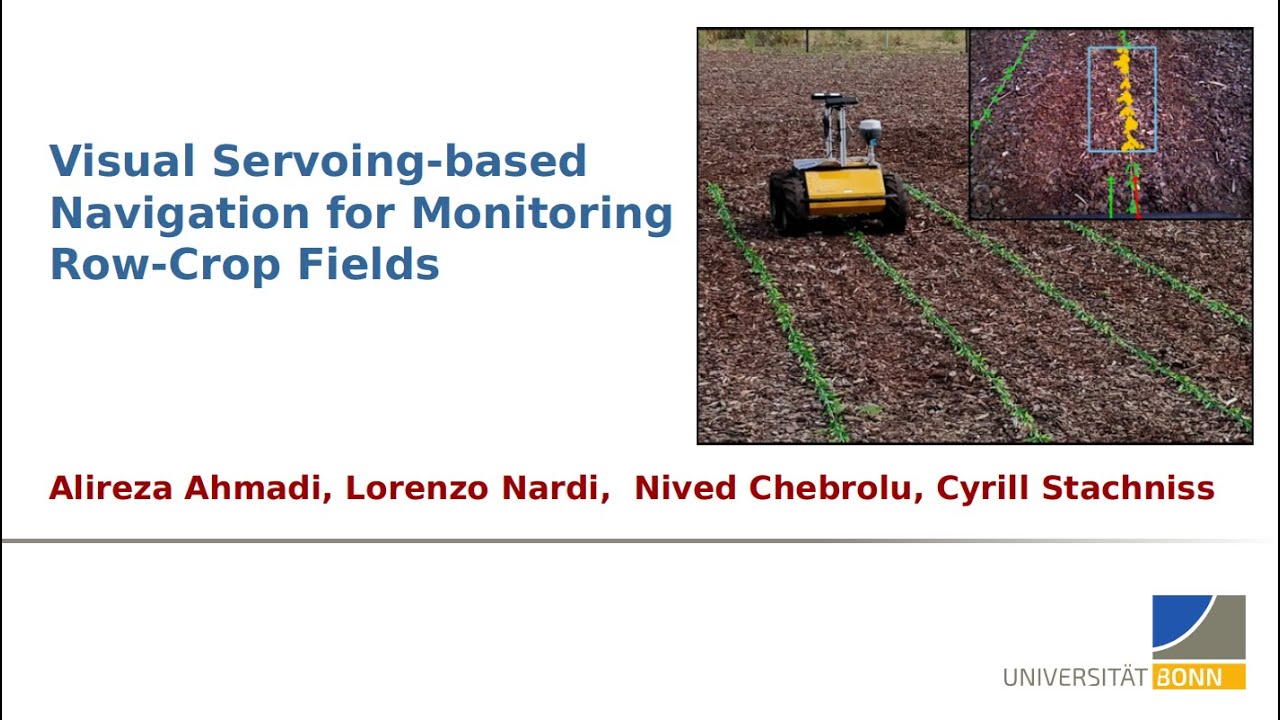
ICRA 2020 Presentation – Visual-Servoing based Navigation for Monitoring Row-Crop Fields
In this project, we propose a framework tailored for navigation in row-crop fields by exploiting the regular crop-row structure present in the fields. Our approach uses only the images from on-board cameras without the need for performing explicit localization or maintaining a map of the field. It allows the robot to follow the crop-rows accurately and handles the switch to the next row seamlessly within the same framework. We implemented our approach using C ++ and ROS and thoroughly tested it in several simulated environments with different shapes and sizes of field Code on Github: https://github.com/PRBonn/visual-crop-row-navigation

ICRA’19: Uncertainty-Aware Path Planning for Navigation on Road Networks Using Augmented MDPs
L. Nardi and C. Stachniss, “Uncertainty-Aware Path Planning for Navigation on Road Networks Using Augmented MDPs ,” in Proc. of the IEEE Intl. Conf. on Robotics & Automation (ICRA) , 2019. http://www.ipb.uni-bonn.de/wp-content/papercite-data/pdf/nardi2019icra-uapp.pdf
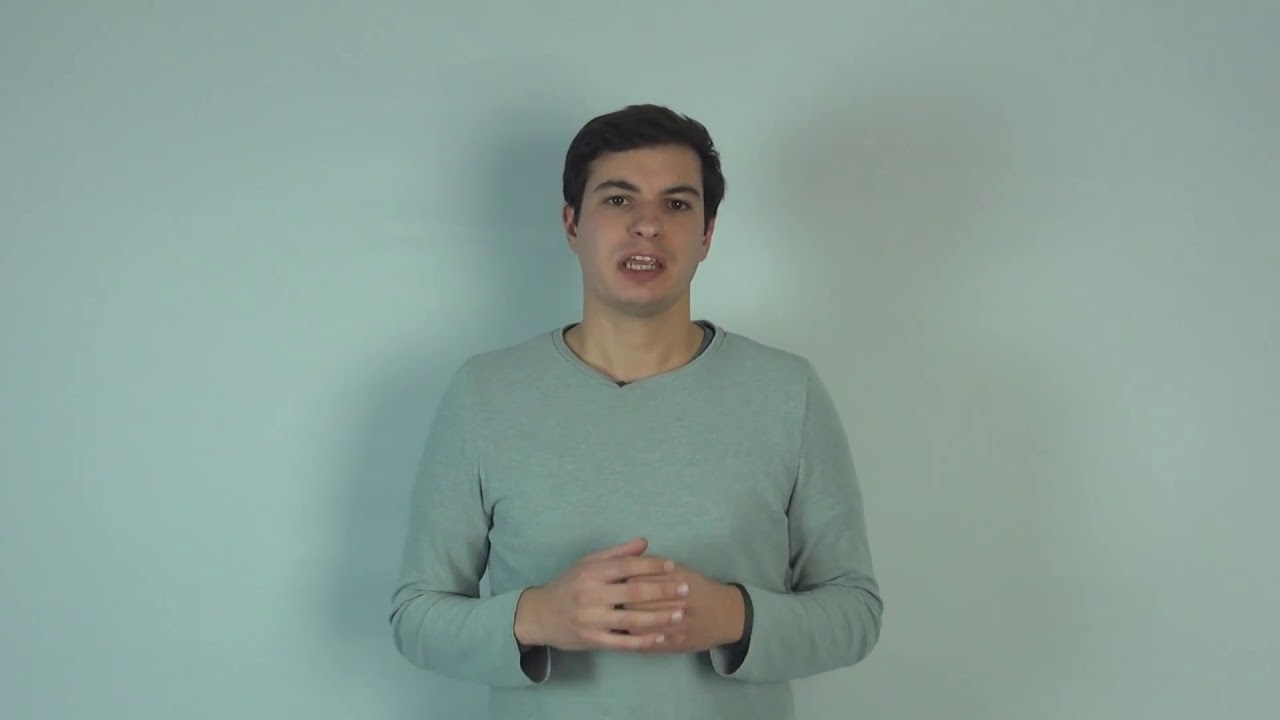
IROS’18 Workshop: Towards Uncertainty-Aware Path Planning for Navigation by Nardi et al.
L. Nardi and C. Stachniss, “Towards Uncertainty-Aware Path Planning for Navigation on Road Networks Using Augmented MDPs,” in 10th Workshop on Planning, Perception and Navigation for Intelligent Vehicles at the IEEE/RSJ Int. Conf. on Intelligent Robots and Systems (IROS), 2018.

Talk by J. Behley on Domain Transfer for Semantic Segmentation of LiDAR Data using DNNs… (IROS’20)
F. Langer, A. Milioto, A. Haag, J. Behley, and C. Stachniss, “Domain Transfer for Semantic Segmentation of LiDAR Data using Deep Neural Networks,” in Proceedings of the IEEE/RSJ Int. Conf. on Intelligent Robots and Systems (IROS), 2020. PDF: https://www.ipb.uni-bonn.de/wp-content/papercite-data/pdf/langer2020iros.pdf
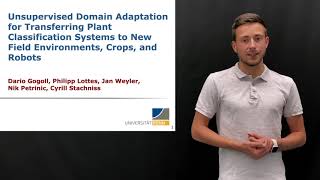
D. Gogoll – Unsupervised Domain Adaptation for Transferring Plant Classification Systems (Trailer)
Watch the full presentation: http://digicrop.de/program/unsupervised-domain-adaptation-for-transferring-plant-classification-systems-to-new-field-environments-crops-and-robots/
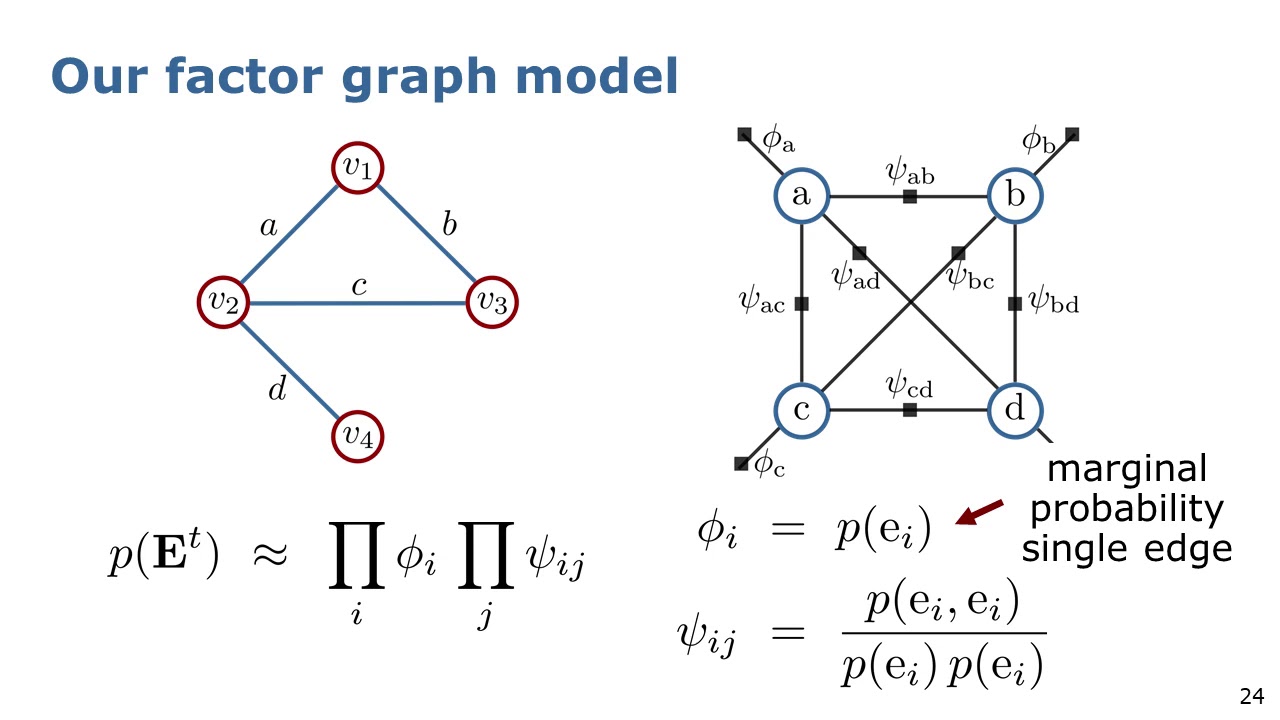
Talk by L. Nardi on Long-Term Robot Navigation in Indoor Environments… (ICRA’20)
ICRA 2020 talk about the paper: L. Nardi and C. Stachniss, “Long-Term Robot Navigation in Indoor Environments Estimating Patterns in Traversability Changes,” in Proceedings of the IEEE Int. Conf. on Robotics & Automation (ICRA), 2020. PDF: https://www.ipb.uni-bonn.de/wp-content/papercite-data/pdf/nardi2020icra.pdf Discussion Slack Channel: https://icra20.slack.com/app_redirect?channel=moa08_1
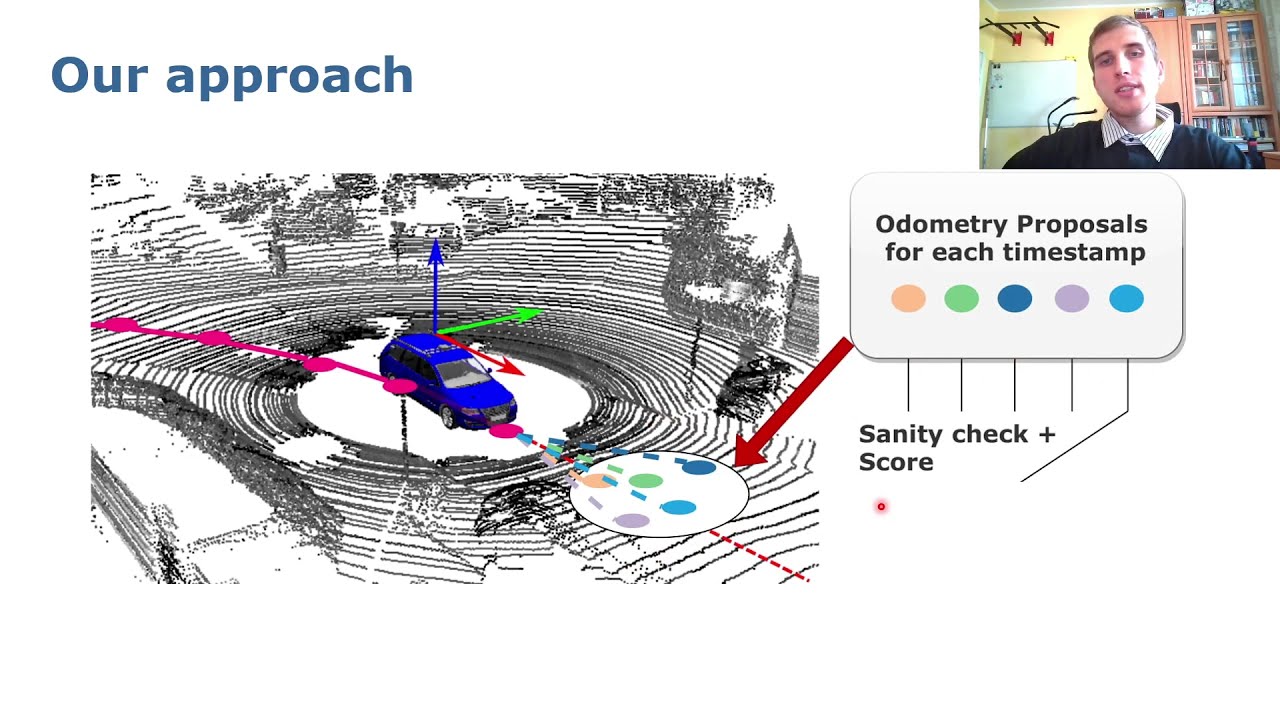
Talk by A. Reinke: Simple But Effective Redundant Odometry for Autonomous Vehicles (ICRA’21)
A. Reinke, X. Chen, and C. Stachniss, “Simple But Effective Redundant Odometry for Autonomous Vehicles,” in Proceedings of the IEEE Int. Conf. on Robotics & Automation (ICRA), 2021. PDF: https://www.ipb.uni-bonn.de/wp-content/papercite-data/pdf/reinke2021icra.pdf Code: https://github.com/PRBonn/MutiverseOdometry #UniBonn #StachnissLab #robotics #autonomouscars #talk
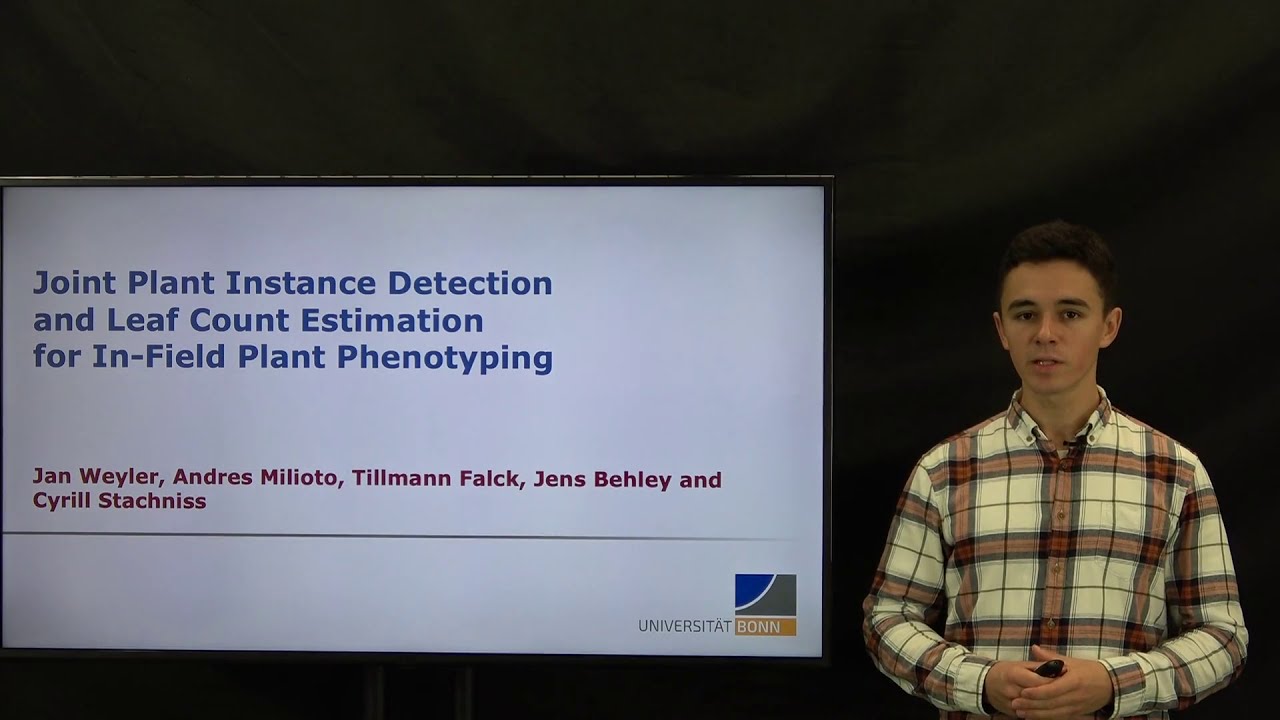
J. Weyler – Joint Plant Instance Detection & Leaf Count Estimation for Plant Phenotyping (Trailer)
Watch the full presentation: http://digicrop.de/program/joint-plant-instance-detection-and-leaf-count-estimation-for-in-field-plant-phenotyping/
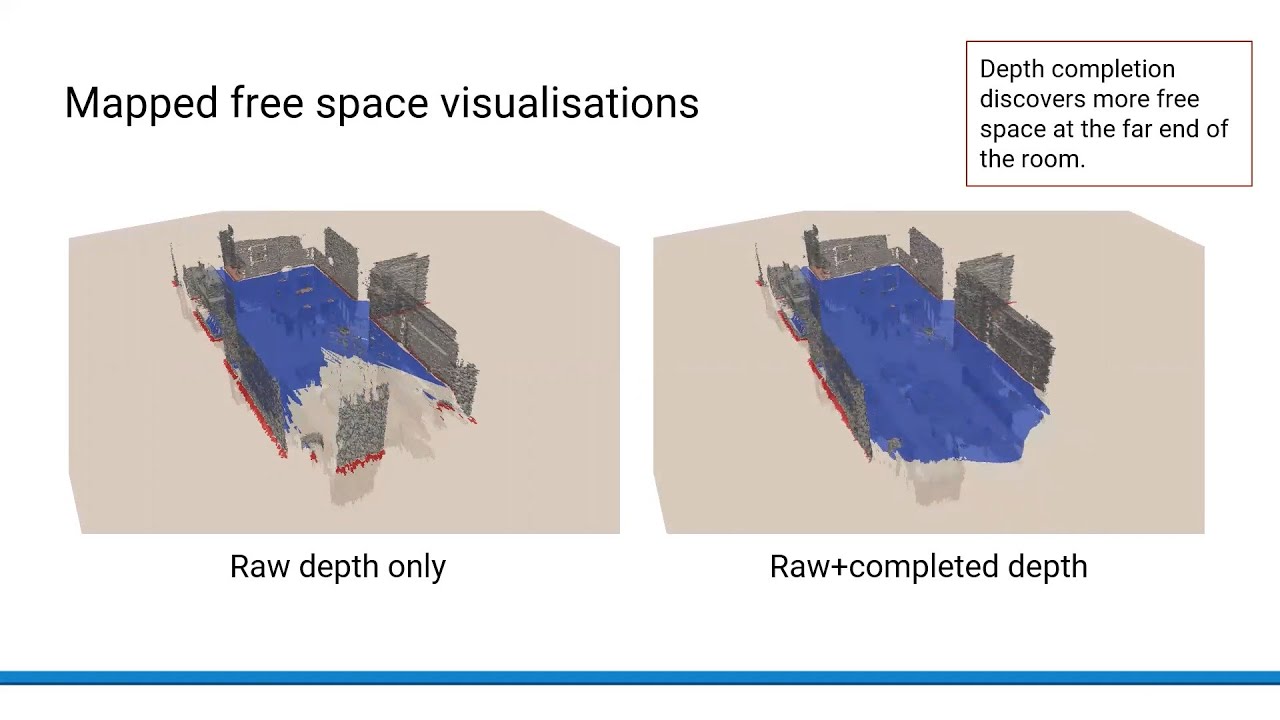
Volumetric Occupancy Mapping With Probabilistic Depth Completion for Robotic Navigation
Volumetric Occupancy Mapping With Probabilistic Depth Completion for Robotic Navigation Marija Popović, Florian Thomas, Sotiris Papatheodorou, Nils Funk, Teresa Vidal-Calleja and Stefan Leutenegger In robotic applications, a key requirement for safe and efficient motion planning is the ability to map obstacle-free space in unknown, cluttered 3D environments. However, commodity-grade RGB-D cameras commonly used for sensing fail to register valid depth values on shiny, glossy, bright, or distant surfaces, leading to missing data in the map. To address this issue, we propose a framework leveraging probabilistic depth completion as an additional input for spatial mapping. We introduce a deep learning architecture providing uncertainty estimates for the depth completion of RGB-D images. Our pipeline exploits the inferred missing depth values and depth uncertainty to complement raw depth images and improve the speed and quality of free space mapping. Evaluations on synthetic data show that our approach maps significantly more correct free space with relatively low error when compared against using raw data alone in different indoor environments; thereby producing more complete maps that can be directly used for robotic navigation tasks. The performance of our framework is validated using real-world data. Paper: https://arxiv.org/abs/2012.03023
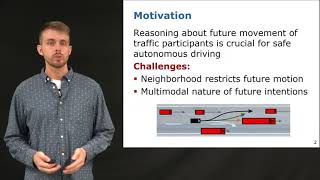
Talk by B. Mersch: Maneuver-based Trajectory Prediction for Self-driving Cars Using … (IROS’21)
B. Mersch, T. Höllen, K. Zhao, C. Stachniss, and R. Roscher, “Maneuver-based Trajectory Prediction for Self-driving Cars Using Spatio-temporal Convolutional Networks,” in Proceedings of the IEEE/RSJ Int. Conf. on Intelligent Robots and Systems (IROS), 2021. Paper: https://www.ipb.uni-bonn.de/wp-content/papercite-data/pdf/mersch2021iros.pdf #UniBonn #StachnissLab #robotics #autonomouscars #neuralnetworks #talk
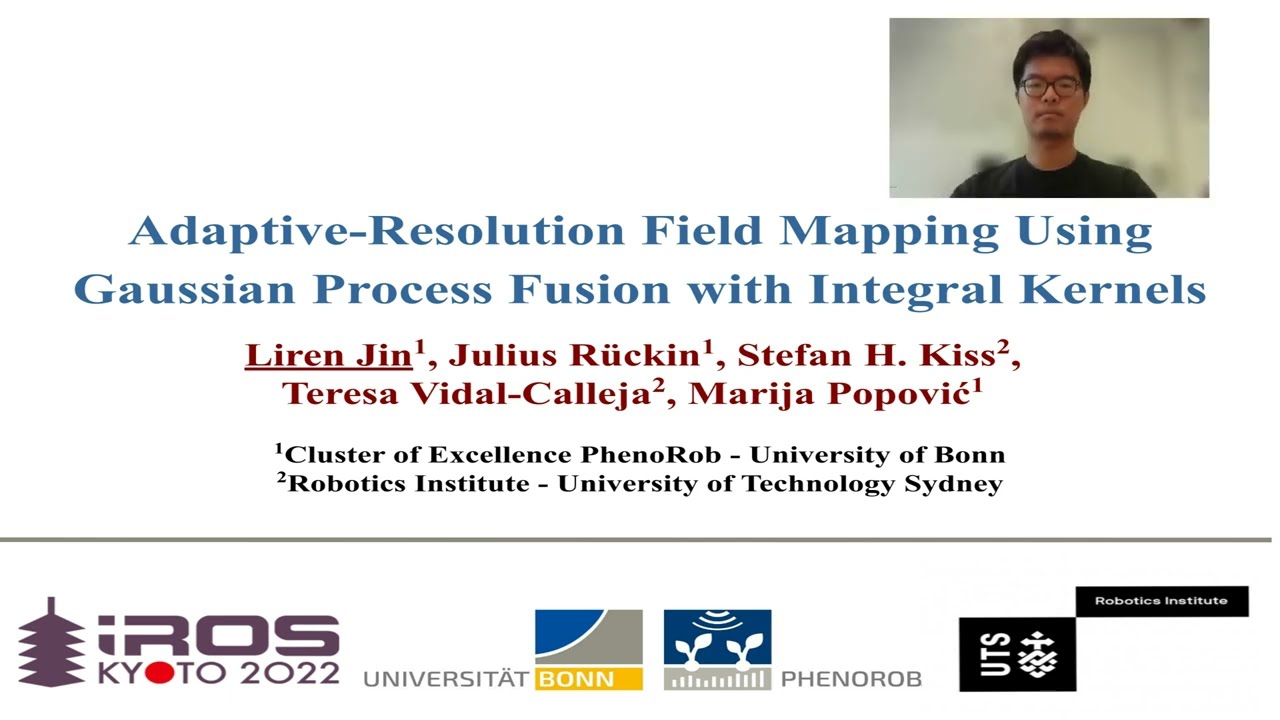
RAL-IROS22: Adaptive-Resolution Field Mapping Using GP Fusion with Integral Kernels, Jin et al.
Jin, L., Rückin J., Kiss, S. H., and Vidal-Calleja, T., and Popović, M., “Adaptive-Resolution Field Mapping Using GP Fusion with Integral Kernels,” IEEE Robotics and Automation Letters (RA-L), vol.7, pp.7471-7478, 2022. doi: 10.1109/LRA.2022.3183797 PDF: https://arxiv.org/abs/2109.14257

IROS22: Informative Path Planning for Active Learning in Aerial Semantic Mapping
Rückin J., Jin, L., Magistri, F., Stachniss, C., and Popović, M., “Informative Path Planning for Active Learning in Aerial Semantic Mapping,” in Proc. of the IEEE/RSJ Int. Conf. on Robotics and Intelligent Systems (IROS), 2022. PDF: https://arxiv.org/abs/2203.01652 Code: https://github.com/dmar-bonn/ipp-al

Talk by L. Lobefaro: Estimating 4D Data Associations Towards Spatial-Temporal Mapping … (IROS’23)
IROS’23 Talk for the paper: L. Lobefaro, M. V. R. Malladi, O. Vysotska, T. Guadagnino, and C. Stachniss, “Estimating 4D Data Associations Towards Spatial-Temporal Mapping of Growing Plants for Agricultural Robots,” in Proc. of the IEEE/RSJ Intl. Conf. on Intelligent Robots and Systems (IROS), 2023. PDF: https://www.ipb.uni-bonn.de/wp-content/papercite-data/pdf/lobefaro2023iros.pdf CODE: https://github.com/PRBonn/plants_temporal_matcher

Trailer: Unsupervised Generation of Labeled Training Images for Crop-Weed Segmentation in New …
Paper trailer for the paper: Y. L. Chong, J. Weyler, P. Lottes, J. Behley, and C. Stachniss, “Unsupervised Generation of Labeled Training Images for Crop-Weed Segmentation in New Fields and on Different Robotic Platforms,” IEEE Robotics and Automation Letters (RA-L), vol. 8, iss. 8, p. 5259–5266, 2023. doi:10.1109/LRA.2023.3293356 PDF: https://www.ipb.uni-bonn.de/wp-content/papercite-data/pdf/chong2023ral.pdf CODE: https://github.com/PRBonn/StyleGenForLabels
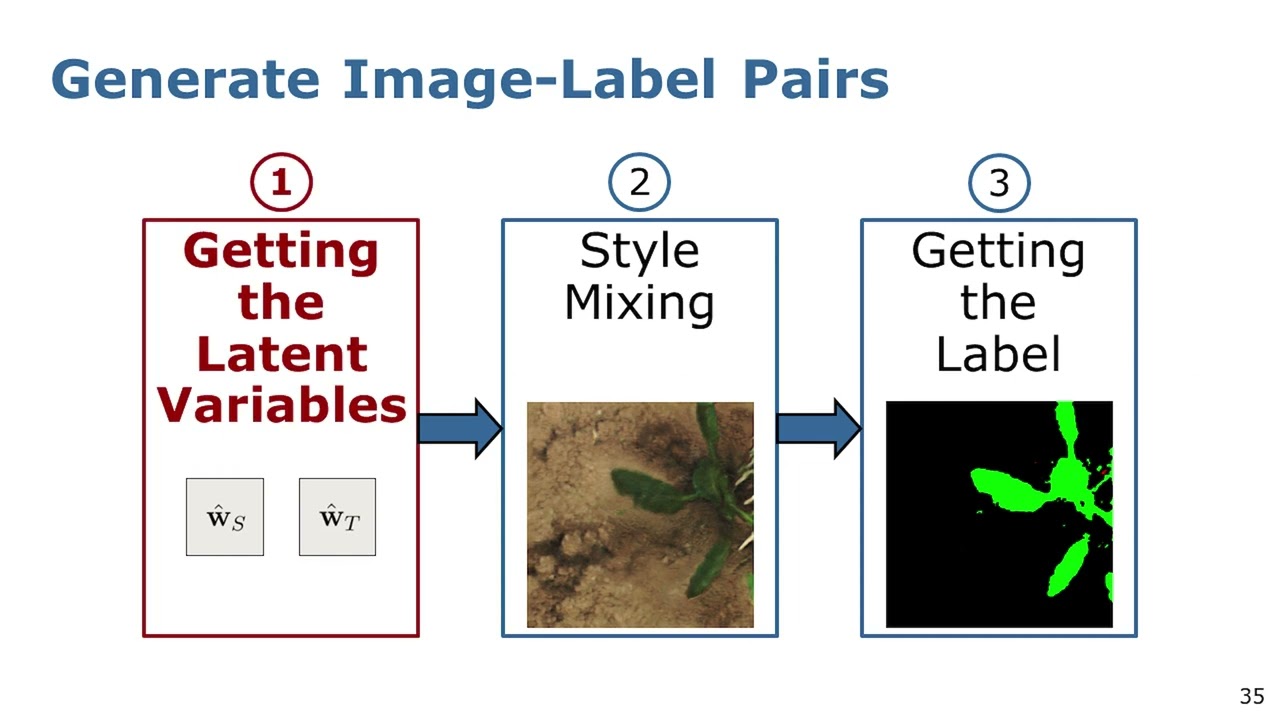
Talk by L. Chong: Unsupervised Generation of Labeled Training Images for Crop-Weed Segmentation…
Talk about the paper: Y. L. Chong, J. Weyler, P. Lottes, J. Behley, and C. Stachniss, “Unsupervised Generation of Labeled Training Images for Crop-Weed Segmentation in New Fields and on Different Robotic Platforms,” IEEE Robotics and Automation Letters (RA-L), vol. 8, iss. 8, p. 5259–5266, 2023. doi:10.1109/LRA.2023.3293356 PDF: https://www.ipb.uni-bonn.de/wp-content/papercite-data/pdf/chong2023ral.pdf CODE: https://github.com/PRBonn/StyleGenForLabels
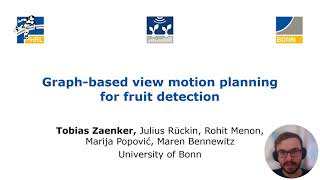
Graph-Based View Motion Planning for Fruit Detection – IROS23 Paper Presentation
Paper presentation by Tobias Zaenker given at IROS 2023. For more details, have a glance at the paper! Title: “Graph-Based View Motion Planning for Fruit Detection” Full paper link: https://arxiv.org/pdf/2303.03048.pdf website: https://www.hrl.uni-bonn.de/Members/tzaenker/tobias-zaenker
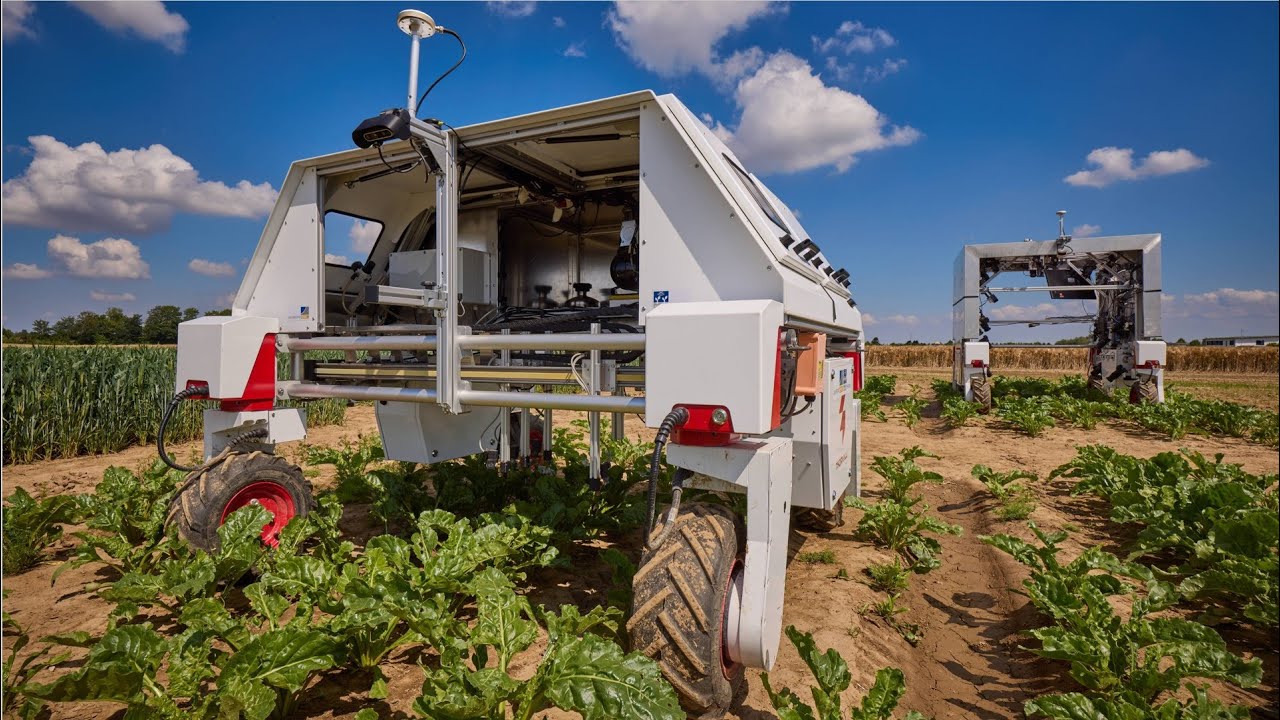
PhenoRob: Research Priorities to Leverage Smart Digital Technologies for Sustainable Crop Production
Agriculture today faces significant challenges that require new ways of thinking, such as smart digital technologies that enable innovative approaches. However, research gaps limit their potential to improve agriculture. In our PhenoRob paper “Research Priorities to Leverage Smart Digital Technologies for Sustainable Crop Production”, Sabine Seidel, Hugo Storm and Lasse Klingbeil outline an interdisciplinary agenda to address the key research gaps and advance sustainability in agriculture. They identify four critical areas: 1. Monitoring to detect weeds and the status of surrounding crops 2. Modelling to predict the yield impact and ecological impacts 3. Decision making by weighing the yield loss against the ecological impact 4. Model uptake, for example policy support to compensate farmers for ecological benefits Closing these gaps requires strong interdisciplinary collaboration. In PhenoRob, this is achieved through five core experiments, seminar and lecture series, and interdisciplinary undergraduate and graduate teaching activities. The paper is available at: H. Storm, S. J. Seidel, L. Klingbeil, F. Ewert, H. Vereecken, W. Amelung, S. Behnke, M. Bennewitz, J. Börner, T. Döring, J. Gall, A. -K. Mahlein, C. McCool, U. Rascher, S. Wrobel, A. Schnepf, C. Stachniss, and H. Kuhlmann, “Research Priorities to Leverage Smart Digital Technologies for Sustainable Crop Production,” European Journal of Agronomy, vol. 156, p. 127178, 2024. doi:10.1016/j.eja.2024.127178 https://www.sciencedirect.com/science/article/pii/S1161030124000996?via%3Dihub