Based on aboveground data collected in the field that display crop development, Core Project 2 identifies unknown correlations towards realizing new expressive features for crop science. Here, the focus is on the link between different growth phases and stress influences such as plant disease, nutrient deficiencies, or drought stress on yield development. We identify key features using machine learning techniques that are validated through experimental design approaches. This leads to new insights into the interpretation of sensor data, as well as support for decision making in practical agriculture or plant breeding.
Research Videos
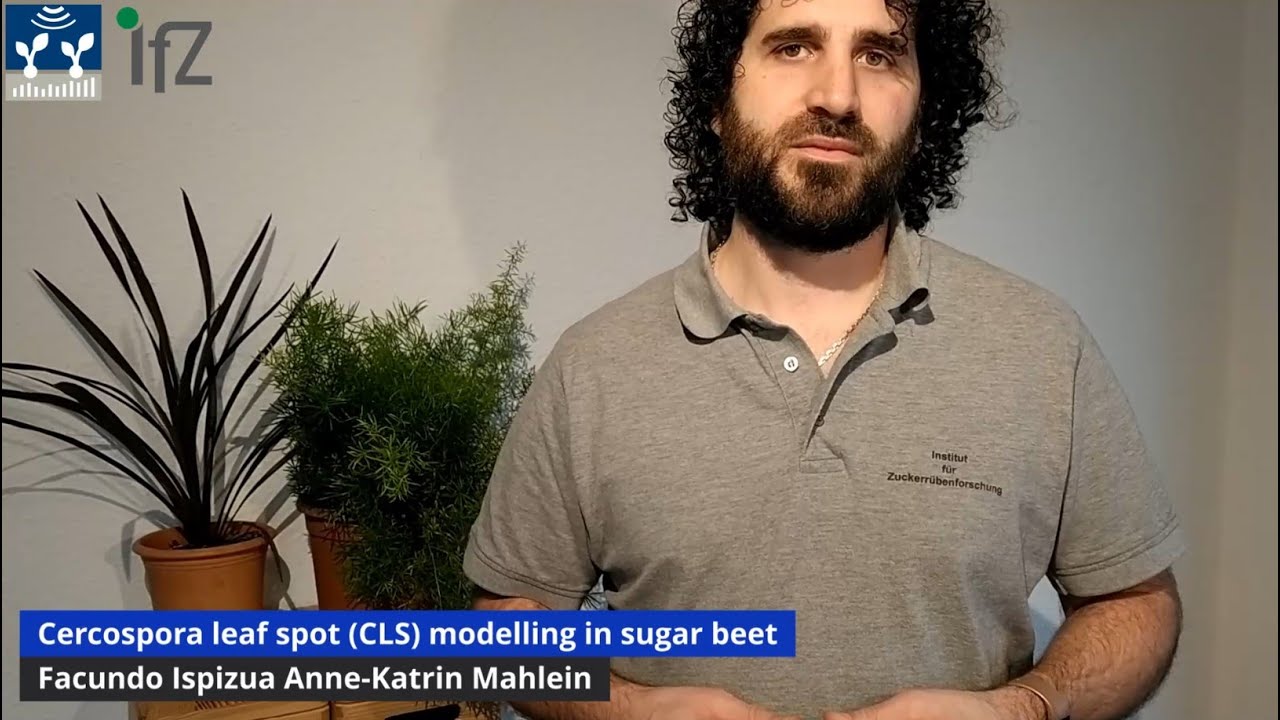
Cercospora Leaf Spot Disease Modelling in Sugar Beet by Optical Sensors and Environmental Data
PhenoRob PhD Student Facundo Ispizua talks about his research within Core Project 2: Relevance Detection of Crop Features.
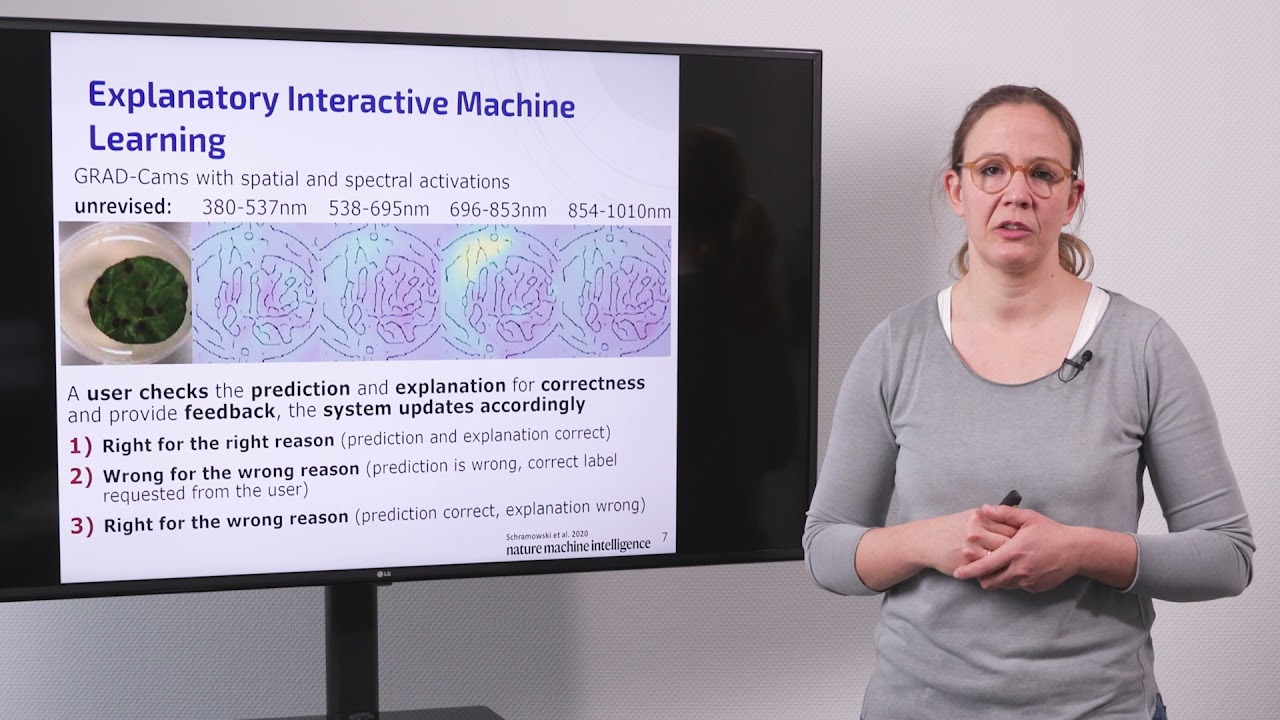
A.-K. Mahlein: Making Deep Neural Networks Right for the Right Scientific Reason…
Prof. Dr. Anne-Katrin Mahlein is Principal Investigator at PhenoRob and Director of the Institute of Sugarbeet Research (IfZ) at the University of Göttingen. Making deep neural networks right for the right scientific reasons by interacting with their explanations. Schramowski, P., Stammer, W., Teso, S. et al. Nat Mach Intell 2, 476–486 (2020). https://doi.org/10.1038/s42256-020-0212-3
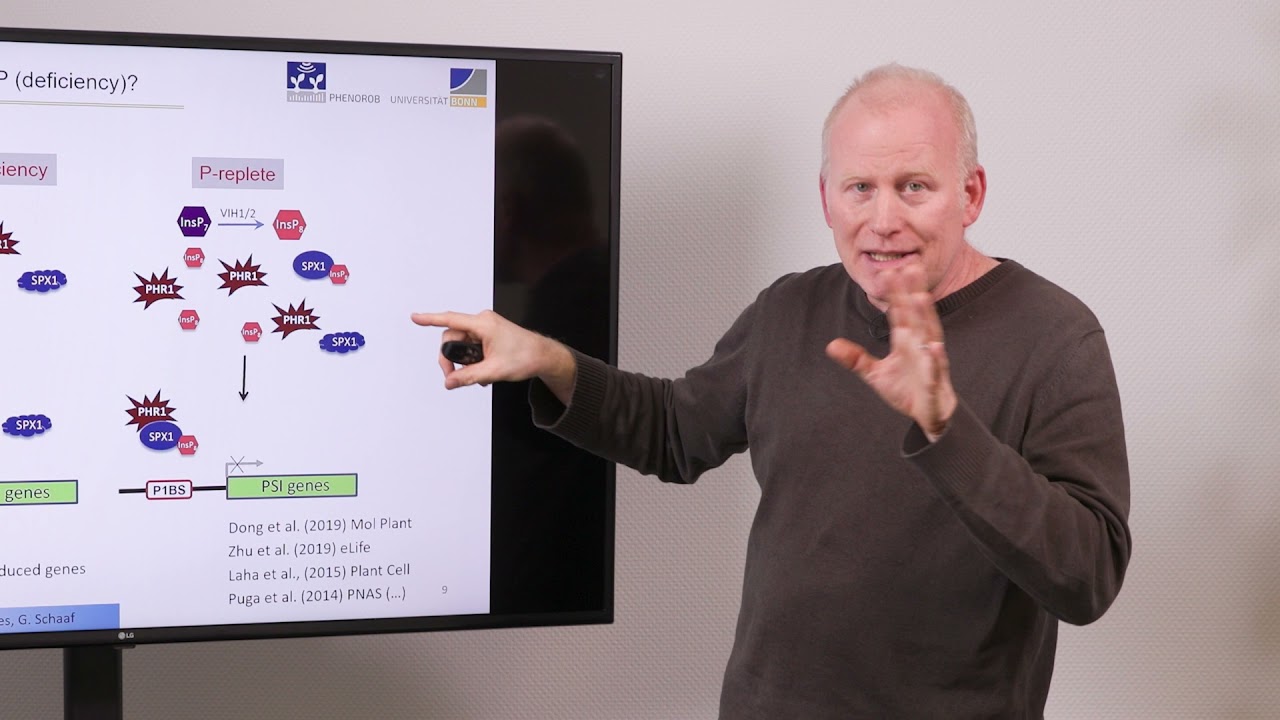
G. Schaaf: ITPK1 is an InsP6/ADP phosphotransferase that controls phosphate signaling in Arabidopsis
Prof. Dr. Gabriel Schaaf is Principal Investigator at PhenoRob and Professor and head of the Ecophysiology of Plant Nutrition Group, Institute of Crop Science and Resource Conservation (INRES), University of Bonn. ITPK1 is an InsP6/ADP phosphotransferase that controls phosphate signaling in Arabidopsis EstherRiemer, Danye Qiu, Debabrata Laha, Robert K.Harmel, Philipp Gaugler, Verena Gaugler, Michael Frei, Mohammad-Reza Hajirezaei, Nargis Parvin Laha, Lukas Krusenbaum, Robin Schneider, Adolfo Saiardi, Dorothea Fiedler, Henning J. Jessen, Gabriel Schaaf, Ricardo F. H. Giehl Molecular Plant Volume 14, Issue 11, 1 November 2021, Pages 1864-1880 https://doi.org/10.1016/j.molp.2021.07.011
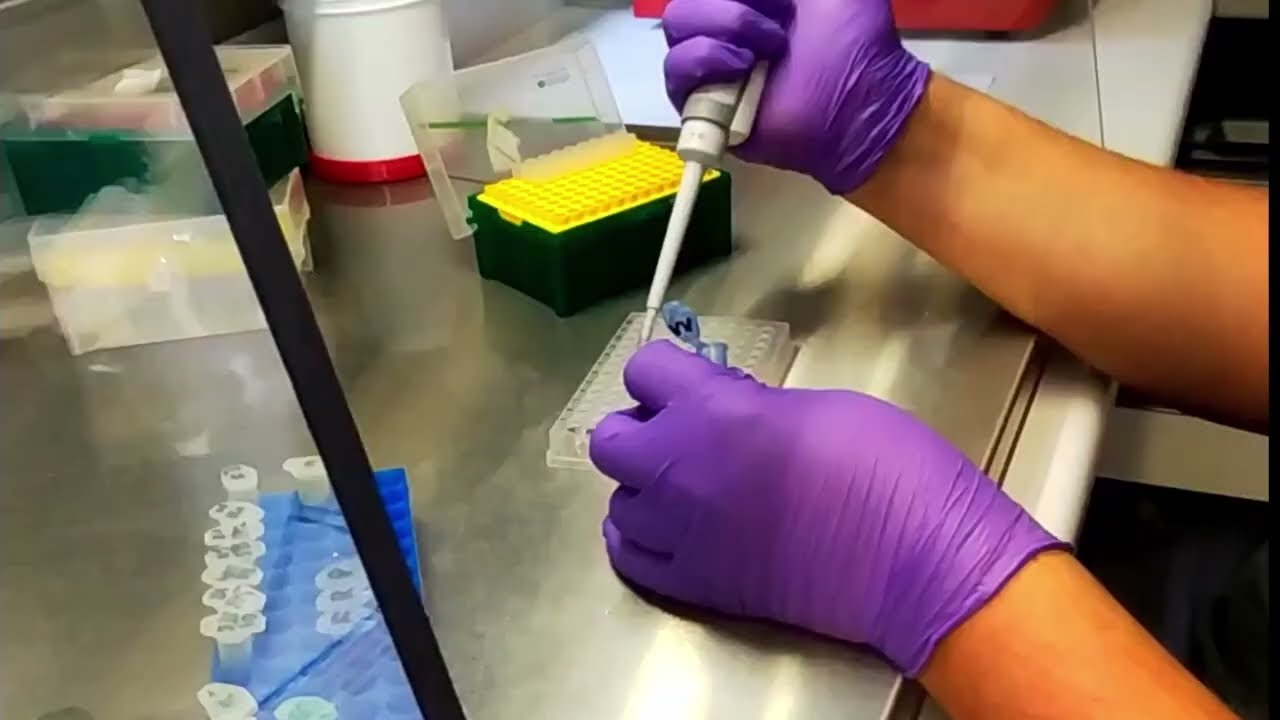
Cercospora leaf spot modeling in sugar beet by Ispizua, Barreto, Günder, Bauckhage & Mahlein
This short paper trailer video is based on the following publication: F. R. Ispizua Yamati, A. Barreto, A. Günder, C. Bauckhage, and A. -K. Mahlein, “Sensing the occurrence and dynamics of Cercospora leaf spot disease using UAV-supported image data and deep learning,” Sugar Industry, vol. 147, iss. 2, pp. 79-86, 2022. Find more info here: https://sugarindustry.info/paper/28345/ https://www.researchgate.net/publication/358243320_Sensing_the_occurrence_and_dynamics_of_Cercospora_leaf_spot_disease_using_UAV-supported_image_data_and_deep_learning
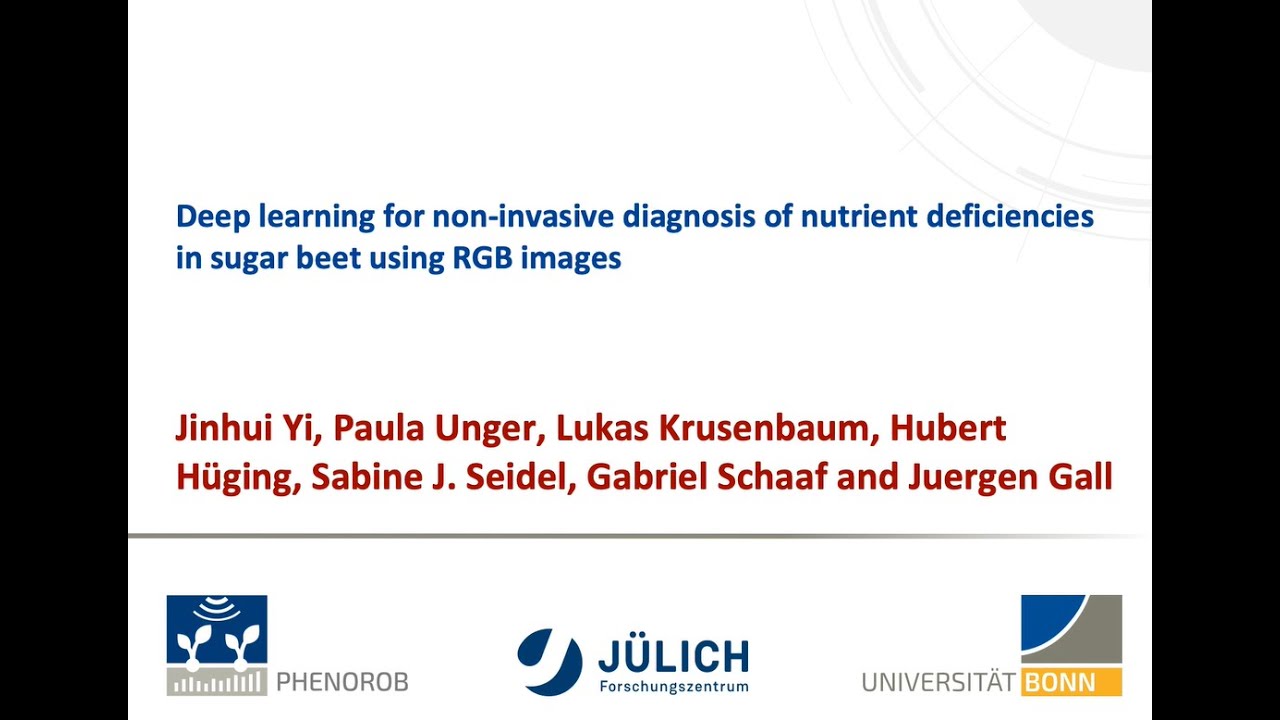
Deep Learning for Non-Invasive Diagnosis of Nutrient Deficiencies in Sugar Beet Using RGB Images
Yi, J.; Krusenbaum, L.; Unger, P.; Hüging, H.; Seidel, S.J.; Schaaf, G.; Gall, J. Deep Learning for Non-Invasive Diagnosis of Nutrient Deficiencies in Sugar Beet Using RGB Images. Sensors 2020, 20, 5893. https://www.mdpi.com/1424-8220/20/20/5893
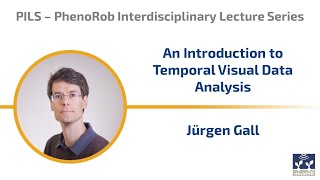
An Introduction to Temporal Visual Data Analysis
Jürgen Gall, professor and head of the Computer Vision Group, Institute of Computer Science at the University of Bonn gives a PhenoRob Interdisciplinary Lecture [PILS] on the topic of temporal visual data analysis.
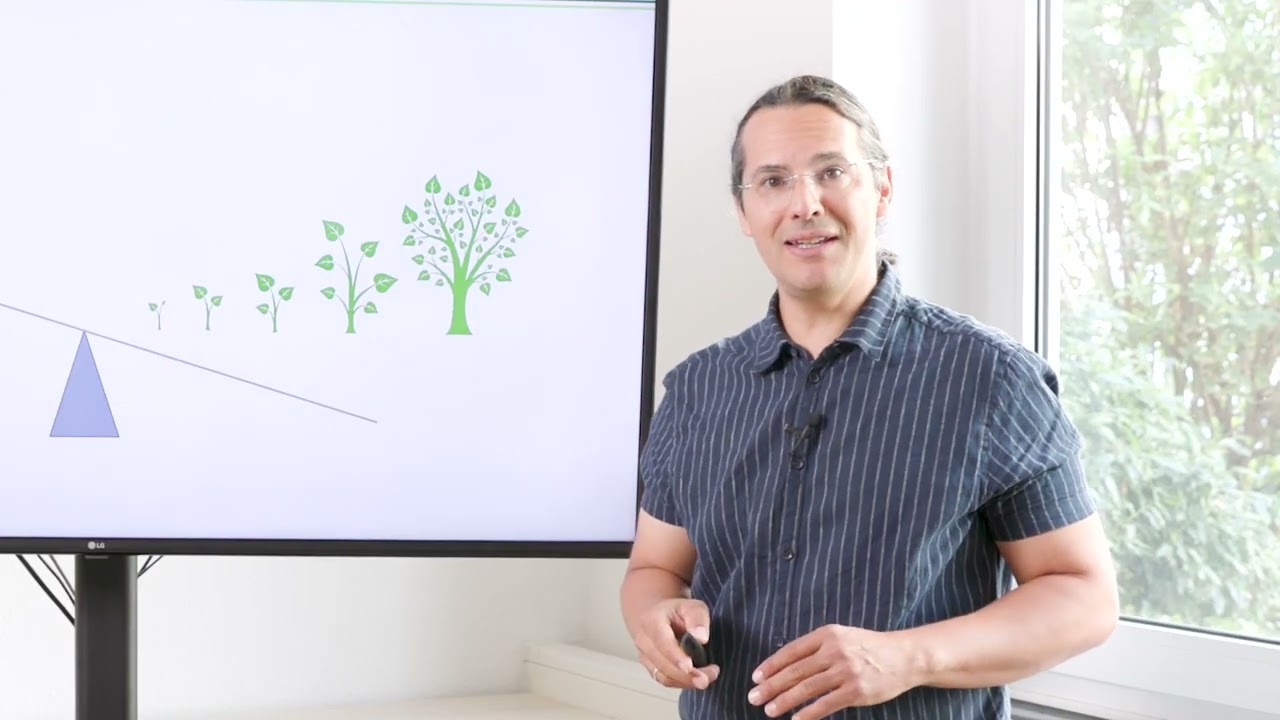
Djamei: Many ways to TOPLESS– manipulation of plant auxin signaling by a cluster of fungal effectors
Prof. Dr. Armin Djamei is Professor of Plant Pathology, Institute of Crop Science and Resource Conservation (INRES) at the University of Bonn. Bindics, J., Khan, M., Uhse, S., Kogelmann, B., Baggely, L., Reumann, D., Ingole, K.D., Stirnberg, A., Rybecky, A., Darino, M., Navarrete, F., Doehlemann, G. and Djamei, A. (2022), Many ways to TOPLESS – manipulation of plant auxin signalling by a cluster of fungal effectors. New Phytologist. https://doi.org/10.1111/nph.18315
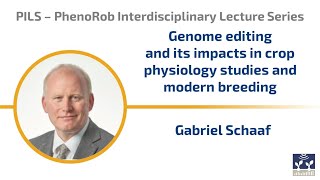
Genome Editing and Its Impacts in Crop Physiology Studies and Modern Breeding
Gabriel Schaaf, Professor and head of the Ecophysiology of Plant Nutrition Group at the Institute of Crop Science and Resource Conservation (INRES), University of Bonn gives a PhenoRob Interdisciplinary Lecture [PILS] on genome editing and its impacts in crop physiology studies and modern breeding
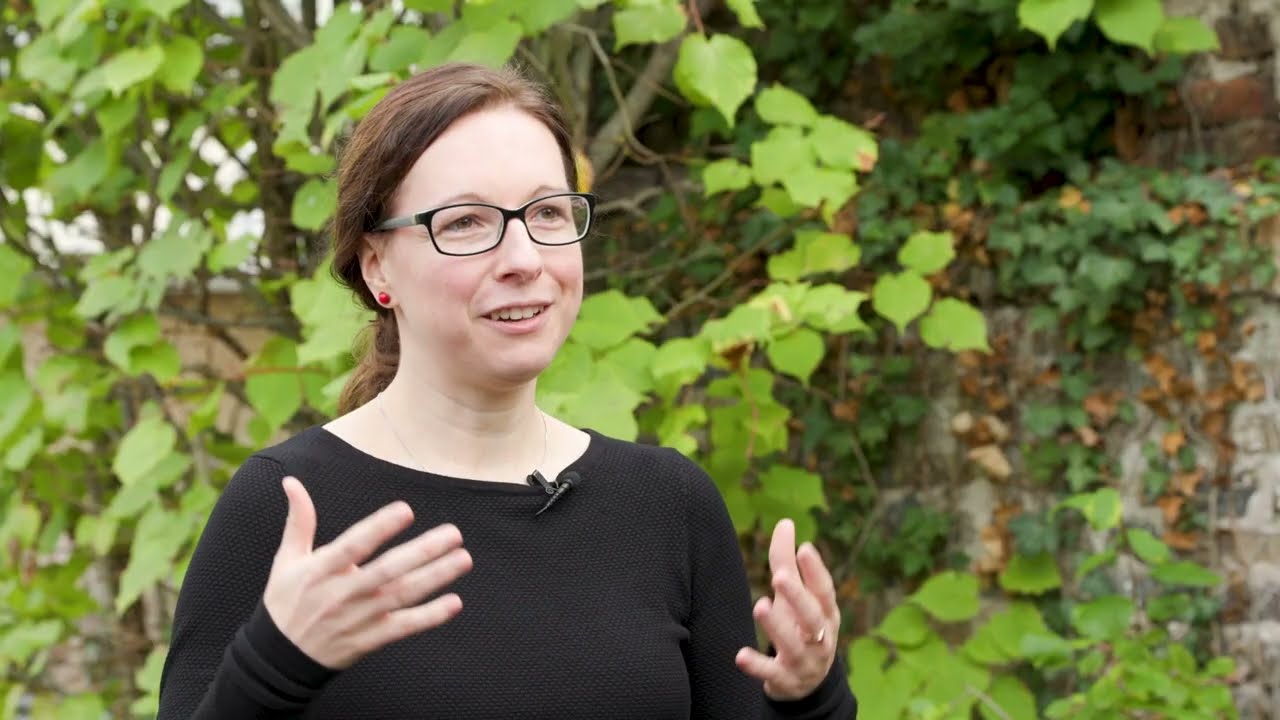
Faces of PhenoRob: Ribana Roscher
In Faces of PhenoRob, we introduce you to some of PhenoRob’s many members: from senior faculty to PhDs, this is your chance to meet them all and learn more about the work they do! In this video, you’ll meet Ribana Roscher, Professor of Data Science for Crop Systems at Forschungszentrum Jülich. https://www.fz-juelich.de/profile/roscher_r
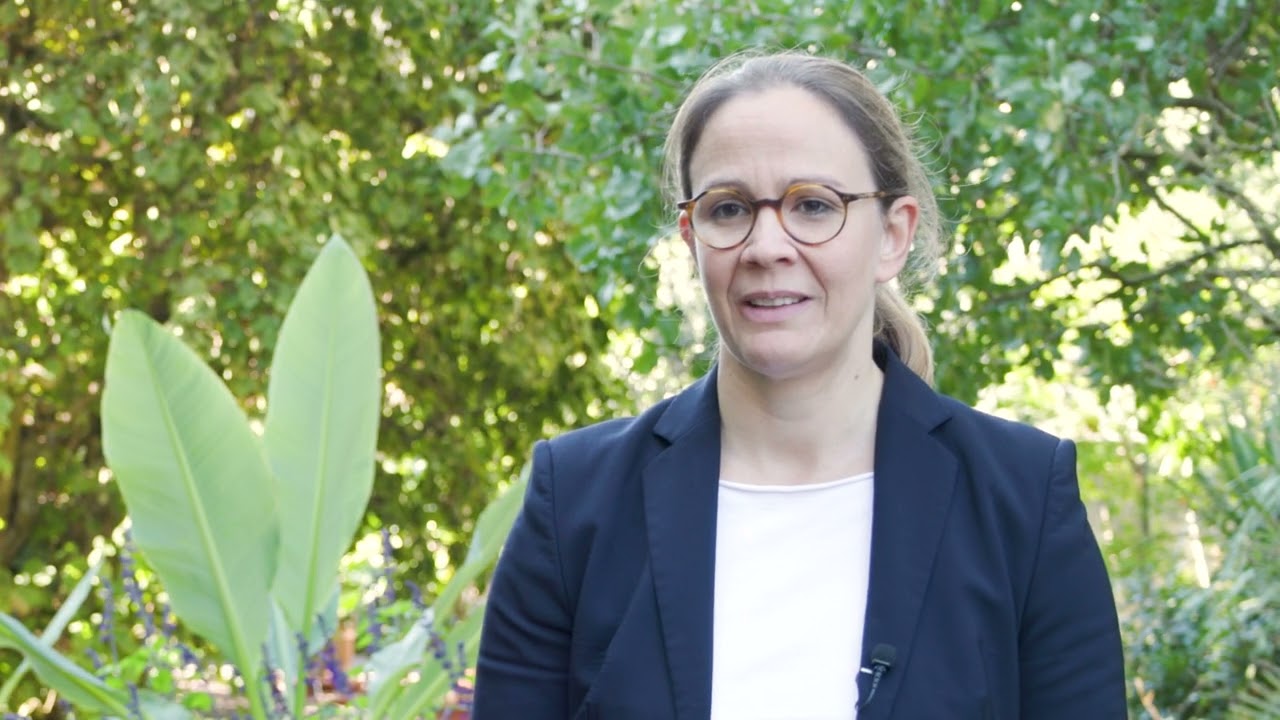
Faces of PhenoRob: Anne-Katrin Mahlein
In Faces of PhenoRob, we introduce you to some of PhenoRob’s many members: from senior faculty to PhDs, this is your chance to meet them all and learn more about the work they do! In this video, you’ll meet Anne-Katrin Mahlein, Director of the Institute of Sugarbeet Research (IfZ), University of Göttingen.
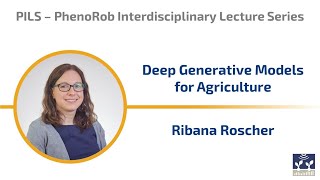
Deep Generative Models for Agriculture
Ribana Roscher, Professor of Data Science for Crop Systems, Institute of Bio- and Geosciences (IBG-2), Forschungszentrum Jülich, and Institute of Geodesy and Geoinformation (IGG), University of Bonn gives a PhenoRob Interdisciplinary Lecture [PILS] on the topic of deep generative models for agriculture.

Faces of PhenoRob: Facundo Ispizua Yamati
In Faces of PhenoRob, we introduce you to some of PhenoRob’s many members: from senior faculty to PhDs, this is your chance to meet them all and learn more about the work they do! In this video, you’ll meet Facundo Ispizua Yamati, PhenoRob PhD student at the Institute of Sugarbeet Research.
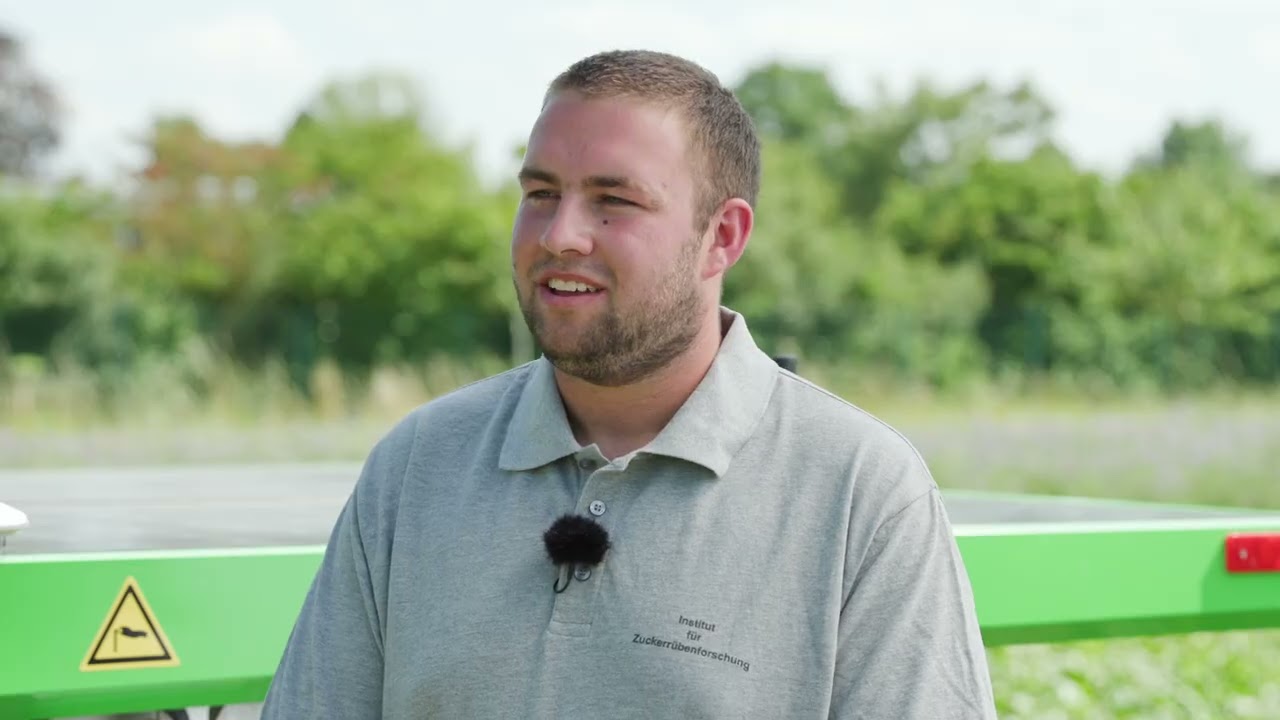
Faces of PhenoRob: Jonathan Eggers
In “Faces of PhenoRob” stellen wir dir einige der vielen Mitglieder von PhenoRob vor. Lernen Sie leitende Institutsmitarbeiter bis hin zu Doktoranden kennen und erfahren Sie mehr über ihre Arbeit! In diesem Video stellen wir Jonathan Eggers vor. Er ist Landwirtschaftstechniker am Institut für Zuckerrübenforschung.

Faces of Phenorob: Justus Detring
In Faces of PhenoRob, we introduce you to some of PhenoRob’s many members: from senior faculty to PhDs, this is your chance to meet them all and learn more about the work they do! In this video, you’ll meet Justus Detring, PhenoRob PhD Student at the Institute of Sugarbeet Research.
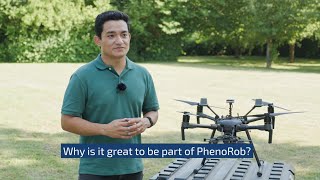
Faces of PhenoRob: Abel Barreto
In Faces of PhenoRob, we introduce you to some of PhenoRob’s many members: from senior faculty to PhDs, this is your chance to meet them all and learn more about the work they do! In this video, you’ll meet Abel Barreto, PhenoRob PhD Student at the Institute of Sugarbeet Research.
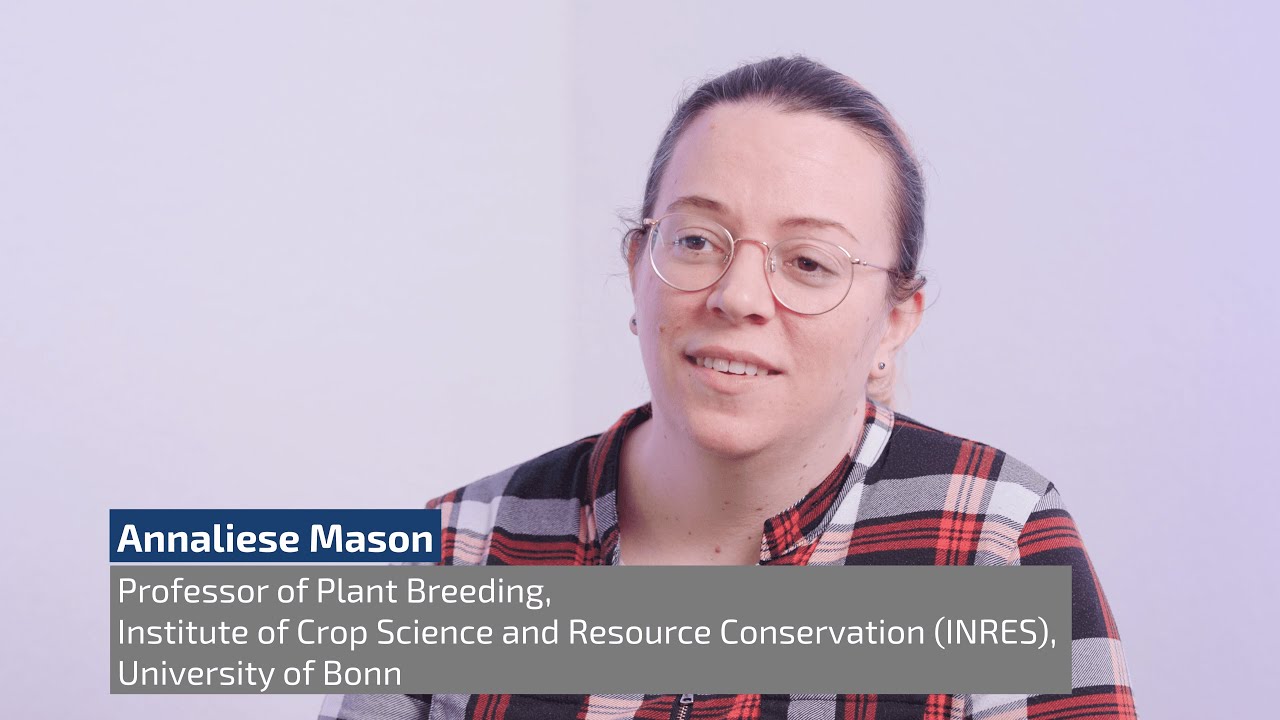
Faces of PhenoRob: Annaliese Mason
In Faces of PhenoRob, we introduce you to some of PhenoRob’s many members: from senior faculty to PhDs, this is your chance to meet them all and learn more about the work they do! In this video you’ll meet Annaliese Mason, Professor and Chair of Plant Breeding at the University of Bonn.

Effect of Different Postharvest Methods on Essential Oil Content and Composition of Mentha Genotypes
Charlotte Hubert-Schöler, PhD student at the Institute of Crop Science and Resource Conservation (INRES), University of Bonn, presents her paper on the “Effect of Different Postharvest Methods on Essential Oil Content and Composition of Three Mentha Genotypes” as part of the “Quality Assessment of Aromatic Plants by Hyperspectral and Mass spectrometric methods (QuAAP)” project. Hubert, C.; Tsiaparas, S.; Kahlert, L.; Luhmer, K.; Moll, M.D.; Passon, M.; Wüst, M.; Schieber, A.; Pude, R. Effect of Different Postharvest Methods on Essential Oil Content and Composition of Three Mentha Genotypes. Horticulturae 2023, 9, 960. https://doi.org/10.3390/horticulturae9090960
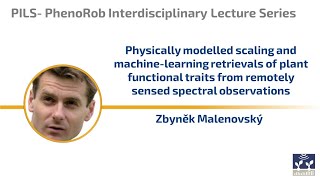
Physically modelled scaling and ML retrievals of plant traits from remotely sensed spectral observ.
Zbyněk Malenovský presents in this PhenoRob Interdisciplinary Lecture Series [PILS] “Physically modelled scaling and machine-learning retrievals of plant functional traits from remotely sensed spectral observations” He is an optical remote sensing and plant eco-physiology scientist based at the University of Bonn.

AGRASIM: a new research facility for simulating the effects of climate change on agroecosystems
Nicolas Brüggemann, Professor for Terrestrial Biogeochemistry at the University of Bonn and Head of the research group “Plant-Soil-Atmosphere Exchange Processes” at the Forschungszentrum Jülich, gives a PhenoRob Interdisciplinary lecture [PILS] on the topic of “AGRASIM: a new research facility at IBG-3 for simulating the effects of climate change on agroecosystems”.

Decision Snippet Features
We show that we can compress random forest models while maintaining their predictive performance by finding Decision Snippet Features. In our presentation at the IEEE International Conference on Pattern Recognition 2021, we show what Decision Snippets are, how you can find them, and which benefits you can expect from Decision Snippets.
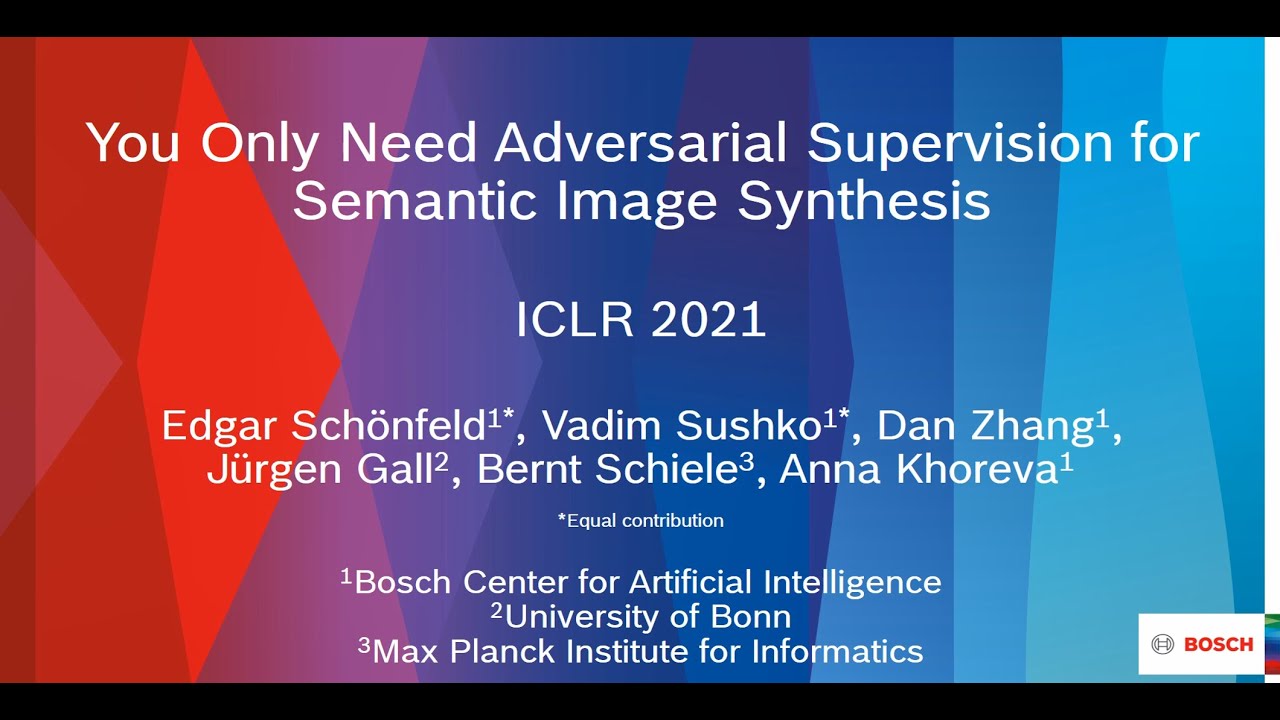
You Only Need Adversarial Supervision for Semantic Image Synthesis, ICLR 2021 (5 min overview)
We introduce OASIS, a novel GAN model for semantic image synthesis that needs only adversarial supervision to achieve state-of-the-art performance. OpenReview: https://openreview.net/forum?id=yvQKLaqNE6M Arxiv: https://arxiv.org/abs/2012.04781 Code: https://github.com/boschresearch/OASIS
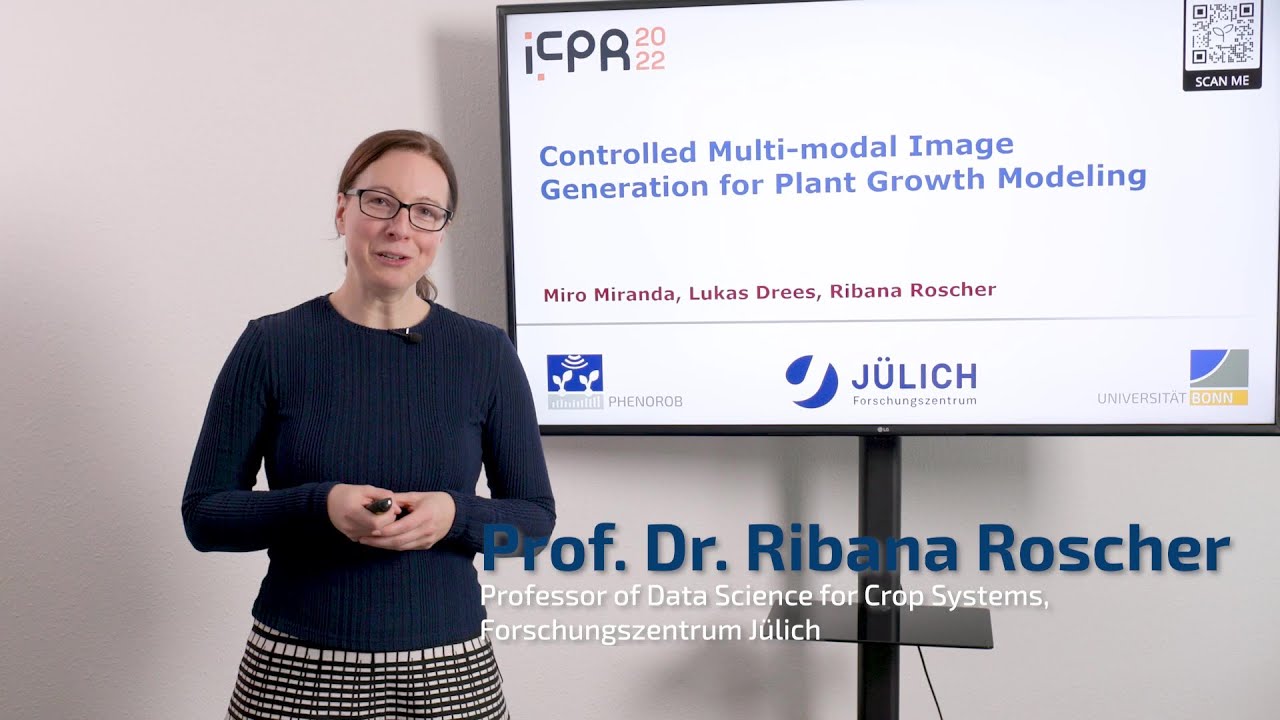
Controlled Multi-modal Image Generation for Plant Growth Modeling
Prof. Dr. Ribana Roscher is Professor of Data Science for Crop Systems, Institute of Bio- and Geosciences (IBG-2) at Forschungszentrum Jülich and Institute of Geodesy and Geoinformation (IGG), University of Bonn M. Miranda, L. Drees and R. Roscher, “Controlled Multi-modal Image Generation for Plant Growth Modeling,” in 2022 26th International Conference on Pattern Recognition (ICPR), Montreal, QC, Canada, 2022 pp. 5118-5124. doi: 10.1109/ICPR56361.2022.9956115

[ECCV 2022 Oral] Adaptive Token Sampling for Efficient Vision Transformers
This is the 5-minute video for our ECCV 2022 paper: “Adaptive Token Sampling for Efficient Vision Transformers” Project Page: https://adaptivetokensampling.github.io/ Paper: https://arxiv.org/pdf/2111.15667.pdf Poster: https://github.com/adaptivetokensampling/adaptivetokensampling.github.io/raw/main/assets/ATS_ECCV_Poster.pdf Code: https://github.com/adaptivetokensampling/ATS Authors: Mohsen Fayyaz*, Soroush Abbasi Koohpayegani*, Farnoush Rezaei-Jafari*, Sunando Sengupta, Hamid-Reza Vaezi-Joze, Eric Sommerlade, Hamed Pirsiavash, Juergen Gall, (* equal contributions). Microsoft, UC Davis, Technical University of Berlin, BIFOLD, University of Bonn Abstract: While state-of-the-art vision transformer models achieve promising results in image classification, they are computationally expensive and require many GFLOPs. Although the GFLOPs of a vision transformer can be decreased by reducing the number of tokens in the network, there is no setting that is optimal for all input images. In this work, we, therefore, introduce a differentiable parameter-free Adaptive Token Sampler (ATS) module, which can be plugged into any existing vision transformer architecture. ATS empowers vision transformers by scoring and adaptively sampling significant tokens. As a result, the number of tokens is not constant anymore and varies for each input image. By integrating ATS as an additional layer within the current transformer blocks, we can convert them into much more efficient vision transformers with an adaptive number of tokens. Since ATS is a parameter-free module, it can be added to the off-the-shelf pre-trained vision transformers as a plug-and-play module, thus reducing their GFLOPs without any additional training. Moreover, due to its differentiable design, one can also train a vision transformer equipped with ATS. We evaluate the efficiency of our module in both image and video classification tasks by adding it to multiple SOTA vision transformers. Our proposed module improves the SOTA by reducing their computational costs (GFLOPs) by 2 times, while preserving their accuracy on the ImageNet, Kinetics-400, and Kinetics-600 datasets.
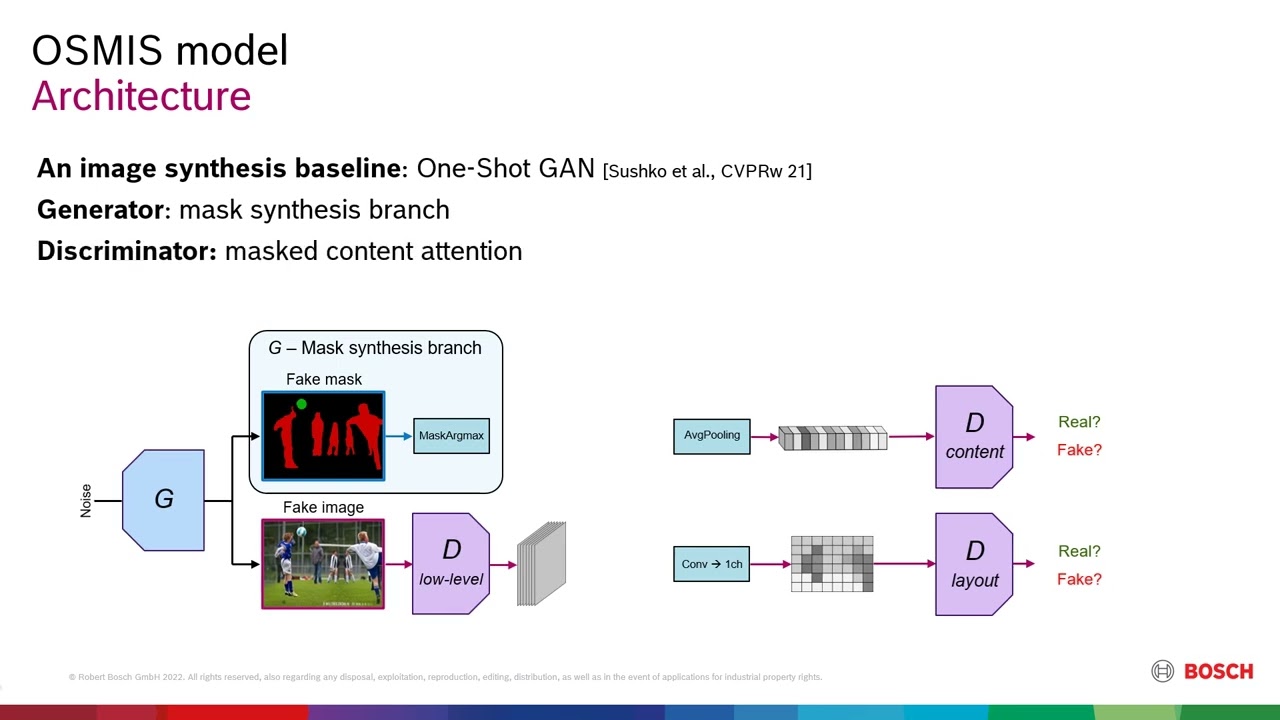
One-Shot Synthesis of Images and Segmentation Masks
Authors: Sushko, Vadim*; Zhang, Dan; Gall, Jürgen; Khoreva, Anna Description: Joint synthesis of images and segmentation masks with generative adversarial networks (GANs) is promising to reduce the effort needed for collecting image data with pixel-wise annotations. However, to learn high-fidelity image-mask synthesis, existing GAN approaches first need a pre-training phase requiring large amounts of image data, which limits their utilization in restricted image domains. In this work, we take a step to reduce this limitation, introducing the task of one-shot image-mask synthesis. We aim to generate diverse images and their segmentation masks given only a single labelled example, and assuming, contrary to previous models, no access to any pre-training data. To this end, inspired by the recent architectural developments of single-image GANs, we introduce our OSMIS model which enables the synthesis of segmentation masks that are precisely aligned to the generated images in the one-shot regime. Besides achieving the high fidelity of generated masks, OSMIS outperforms state-of-the-art single-image GAN models in image synthesis quality and diversity. In addition, despite not using any additional data, OSMIS demonstrates an impressive ability to serve as a source of useful data augmentation for one-shot segmentation applications, providing performance gains that are complementary to standard data augmentation techniques. Code is available at https://github.com/boschresearch/one-shot-synthesis.
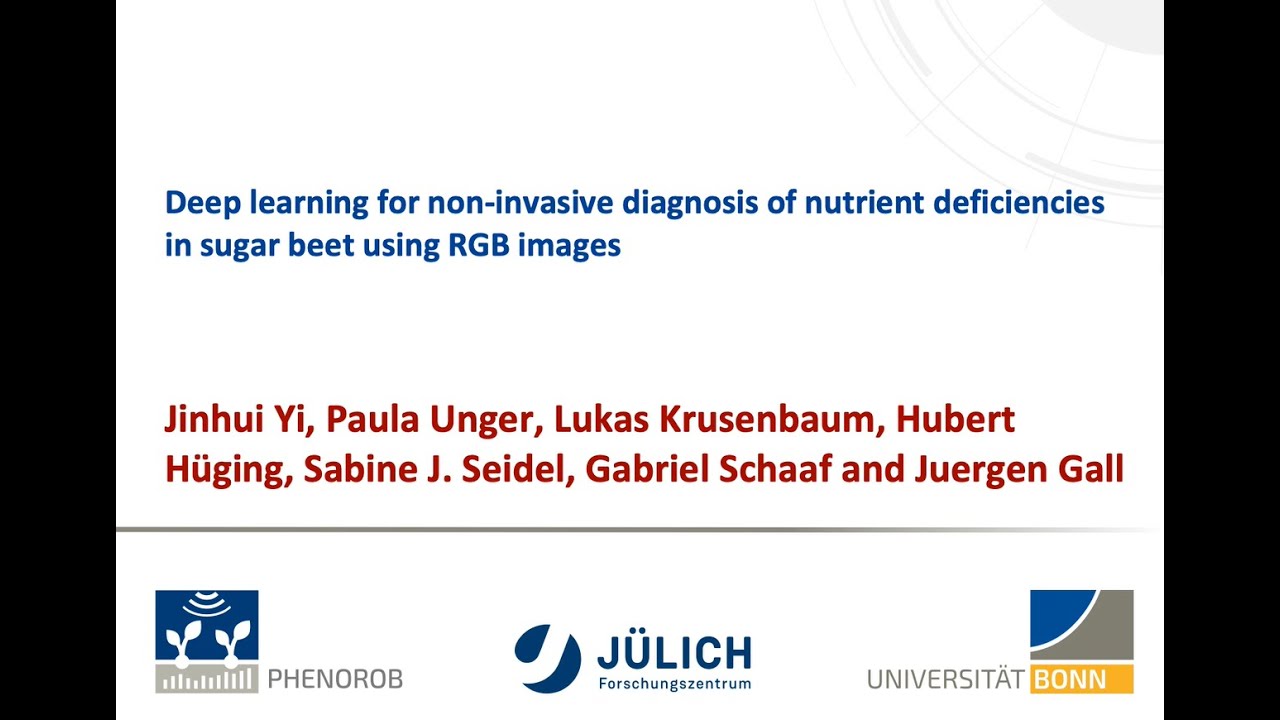
J. Yi – Deep learning for non-invasive diagnosis of nutrient deficiencies in sugar beet
International Conference on Digital Technologies for Sustainable Crop Production (DIGICROP 2020) • November 1-10, 2020 • http://digicrop.de/
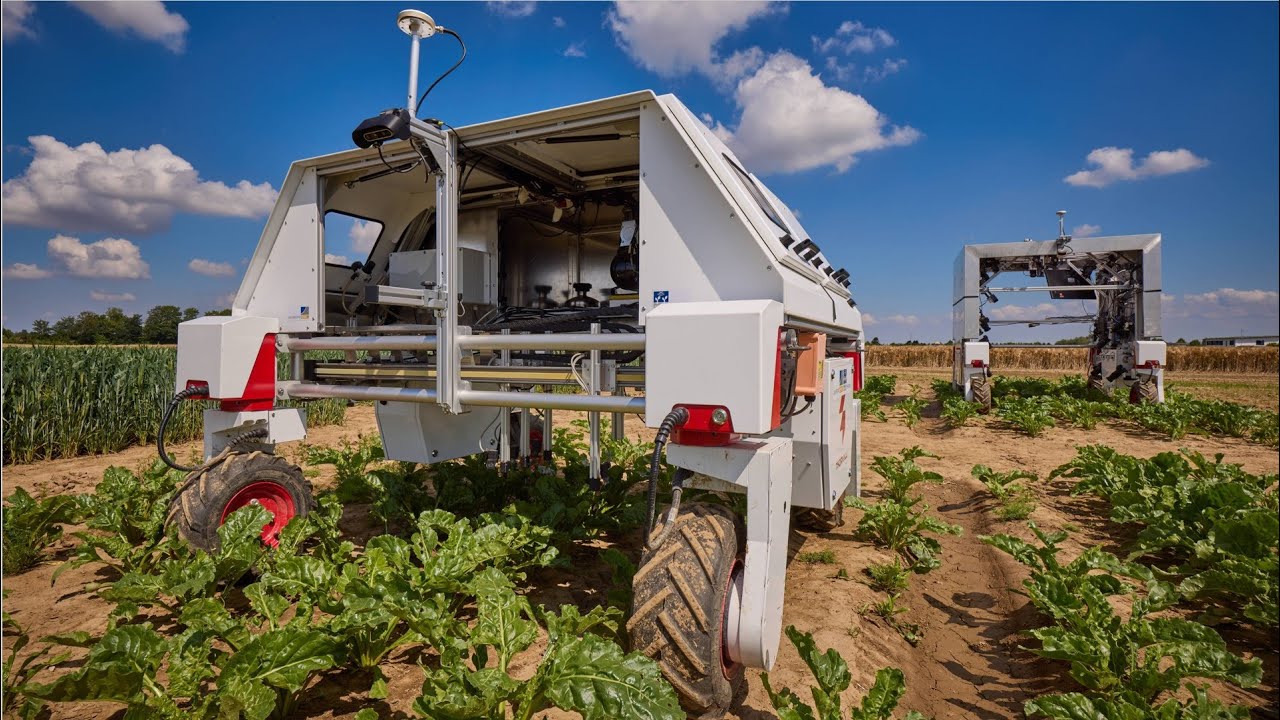
PhenoRob: Research Priorities to Leverage Smart Digital Technologies for Sustainable Crop Production
Agriculture today faces significant challenges that require new ways of thinking, such as smart digital technologies that enable innovative approaches. However, research gaps limit their potential to improve agriculture. In our PhenoRob paper “Research Priorities to Leverage Smart Digital Technologies for Sustainable Crop Production”, Sabine Seidel, Hugo Storm and Lasse Klingbeil outline an interdisciplinary agenda to address the key research gaps and advance sustainability in agriculture. They identify four critical areas: 1. Monitoring to detect weeds and the status of surrounding crops 2. Modelling to predict the yield impact and ecological impacts 3. Decision making by weighing the yield loss against the ecological impact 4. Model uptake, for example policy support to compensate farmers for ecological benefits Closing these gaps requires strong interdisciplinary collaboration. In PhenoRob, this is achieved through five core experiments, seminar and lecture series, and interdisciplinary undergraduate and graduate teaching activities. The paper is available at: H. Storm, S. J. Seidel, L. Klingbeil, F. Ewert, H. Vereecken, W. Amelung, S. Behnke, M. Bennewitz, J. Börner, T. Döring, J. Gall, A. -K. Mahlein, C. McCool, U. Rascher, S. Wrobel, A. Schnepf, C. Stachniss, and H. Kuhlmann, “Research Priorities to Leverage Smart Digital Technologies for Sustainable Crop Production,” European Journal of Agronomy, vol. 156, p. 127178, 2024. doi:10.1016/j.eja.2024.127178 https://www.sciencedirect.com/science/article/pii/S1161030124000996?via%3Dihub